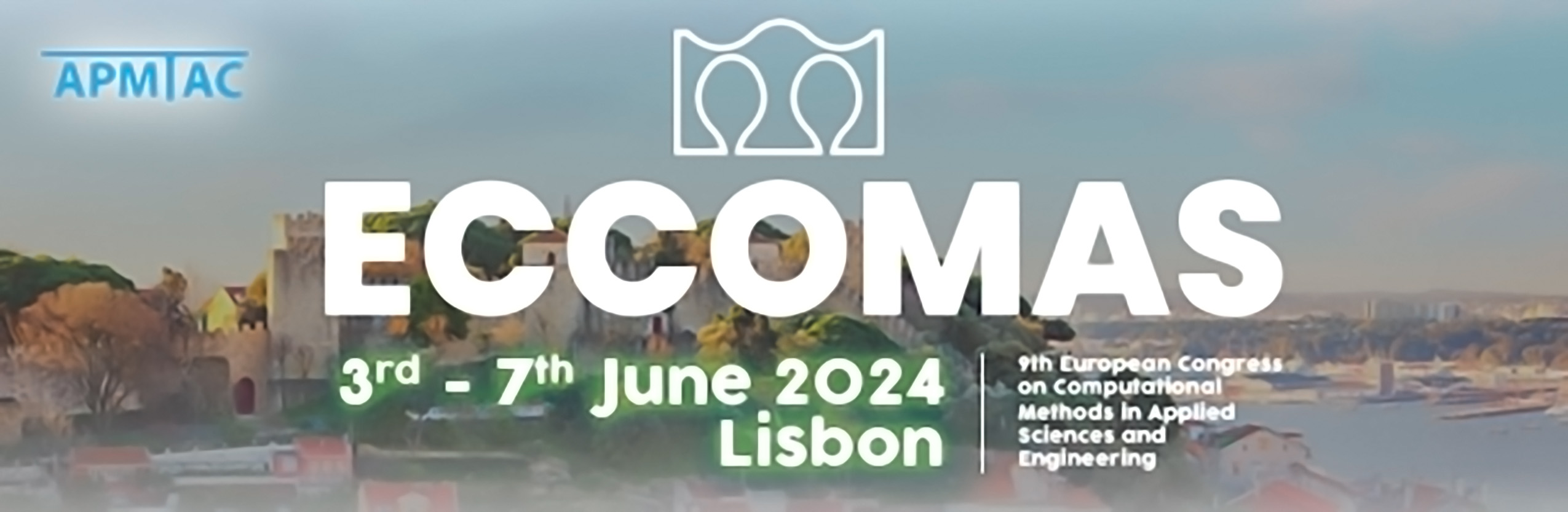
Data-Driven Closures for Explicit Large Eddy Simulations
Please login to view abstract download link
Data from direct numerical simulations (DNS) of turbulent flows are commonly used to train neural network-based models as subgrid closures for Large-Eddy Simulations (LES). In our investigations we used both convolutional and deep networks trained on local and non-local DNS velocity gradients to predict LES closure; specifically we compared prediction of either the sub grid stresses, the divergence of the stresses or equivalent isotropic eddy-viscosity models. The results obtained with various combinations are largely in line with what reported in the literature. However, models with low a priori accuracy have been observed to fortuitously provide better a posteriori results than models with high a priori accuracy. This anomaly can be traced to a dataset shift in the learning problem, arising from inconsistent filtering in the training and testing stages. We propose a resolution to this issue that uses explicit filtering of the nonlinear advection term in the large-eddy simulation momentum equations, to control aliasing errors. Within the context of explicitly-filtered LES, we develop neural network-based models for which a priori accuracy is a good predictor of a posteriori performance. We evaluate the proposed method in a large-eddy simulation of a turbulent flow in a plane channel. Our findings show that an explicitly-filtered large–eddy simulation with a filter-to-grid ratio of 2 suffciently controls the numerical errors so as to allow for accurate and stable simulations.