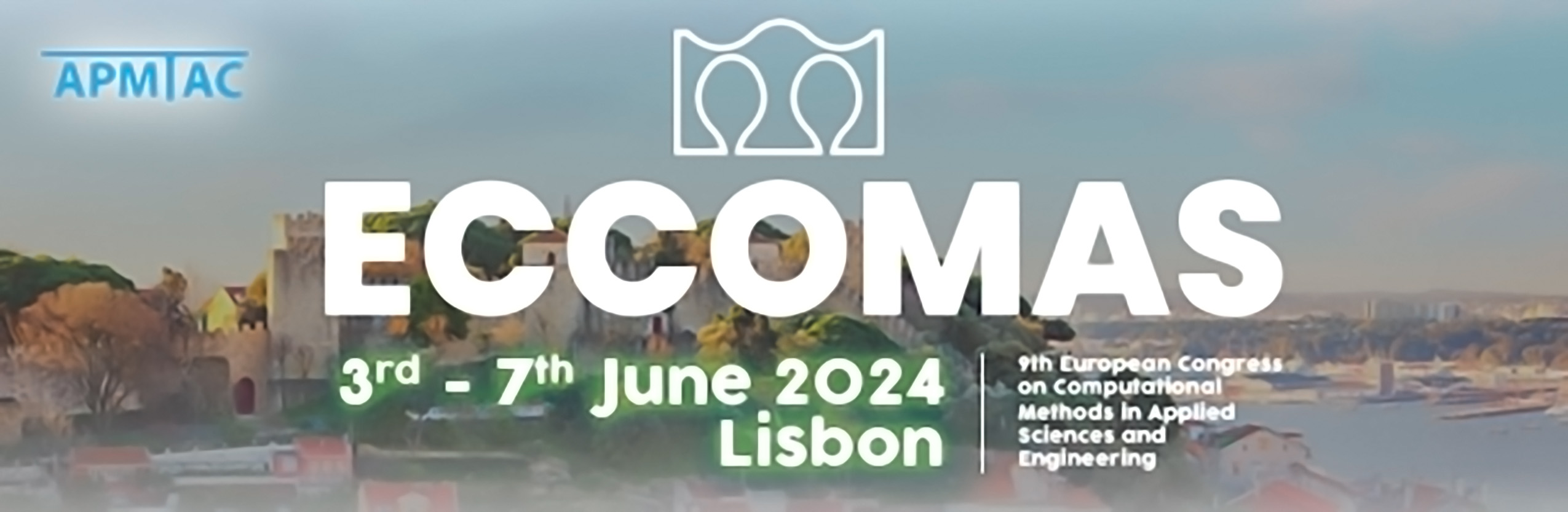
Reduced Basis Approximations of Parameterized Dynamical Partial Differential Equations via Neural Networks
Please login to view abstract download link
Projection-based reduced order models are effective at approximating parameter-dependent differential equations that are parametrically separable. When parametric separability is not satisfied, which occurs in both linear and nonlinear problems, projection-based methods fail to adequately reduce the computational complexity. Devising alternative reduced order models is crucial for obtaining efficient and accurate approximations to expensive high-fidelity models. In this work, we develop a time-stepping procedure for dynamical parameter-dependent problems, in which a neural-network is trained to propagate the coefficients of a reduced basis expansion. This results in an online stage with a computational cost independent of the size of the underlying problem. We demonstrate our method on several parabolic partial differential equations, including a problem that is not parametrically separable.