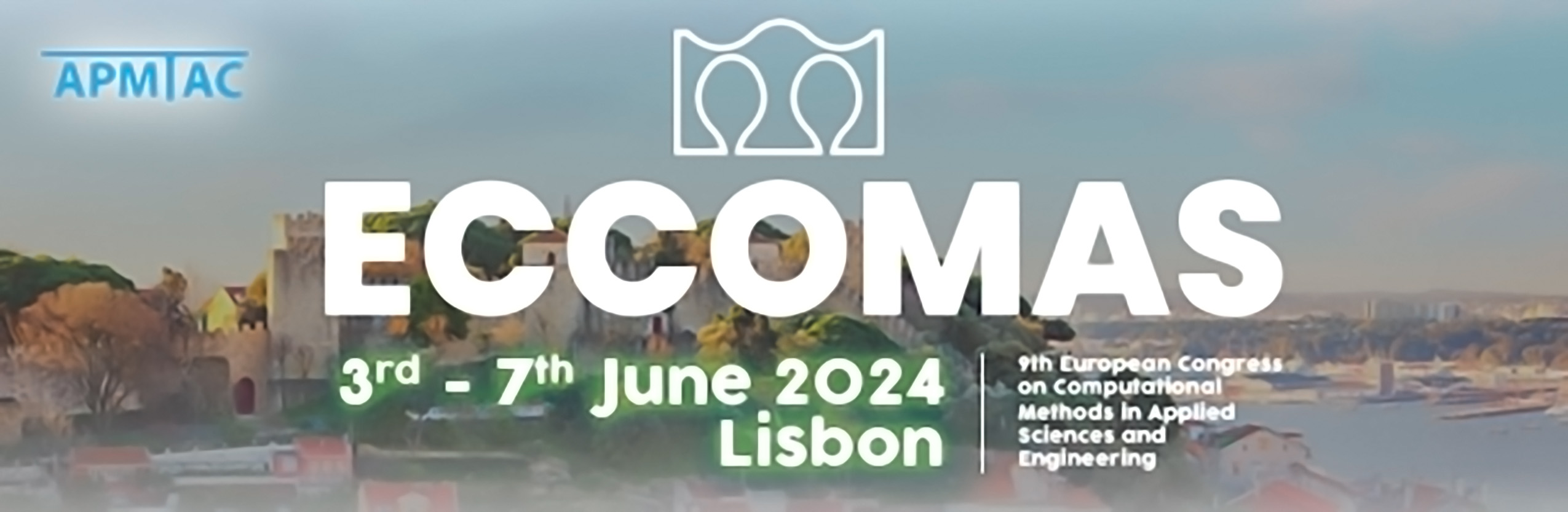
Symmetry-enforcing neural networks for constitutive modeling
Please login to view abstract download link
The use of machine learning techniques to homogenize the effective behavior of arbitrary microstructures has been shown to be not only efficient but also accurate. In a recent work, we demonstrated how to combine state-of-the-art micromechanical modeling and advanced machine learning techniques to homogenize complex microstructures exhibiting non-linear and history dependent behaviors[1]. The resulting homogenized model, which we termed smart constitutive law (SCL), enables the adoption of microstructurally informed constitutive laws into finite element solvers at a fraction of the computational cost required by traditional concurrent multiscale approaches. In this work, we expand the capabilities of SCLs by introducing a novel methodology that enforces material symmetries at the neuron level, applicable across various neural network architectures. Our approach utilizes tensor-based features in neural networks, facilitating the concise and accurate representation of symmetry-preserving operations. We provide details on the construction of these tensor-based neural networks and their application in learning constitutive laws for both elastic and inelastic materials. We demonstrate the superiority of this approach over traditional neural networks in scenarios with limited data and strong symmetries, through comprehensive testing on various materials, including isotropic neo-Hookean materials and tensegrity lattice metamaterials. We conclude our work by discussing the potential of this methodology to discover symmetry bases in materials and outlining future research directions. References [1] Logarzo, H.J., Capuano, G., and Rimoli, J.J. Smart constitutive laws: Inelastic homogenization through machine learning. Computer Methods in Applied Mechanics and Engineering, (2021) 373:113482.