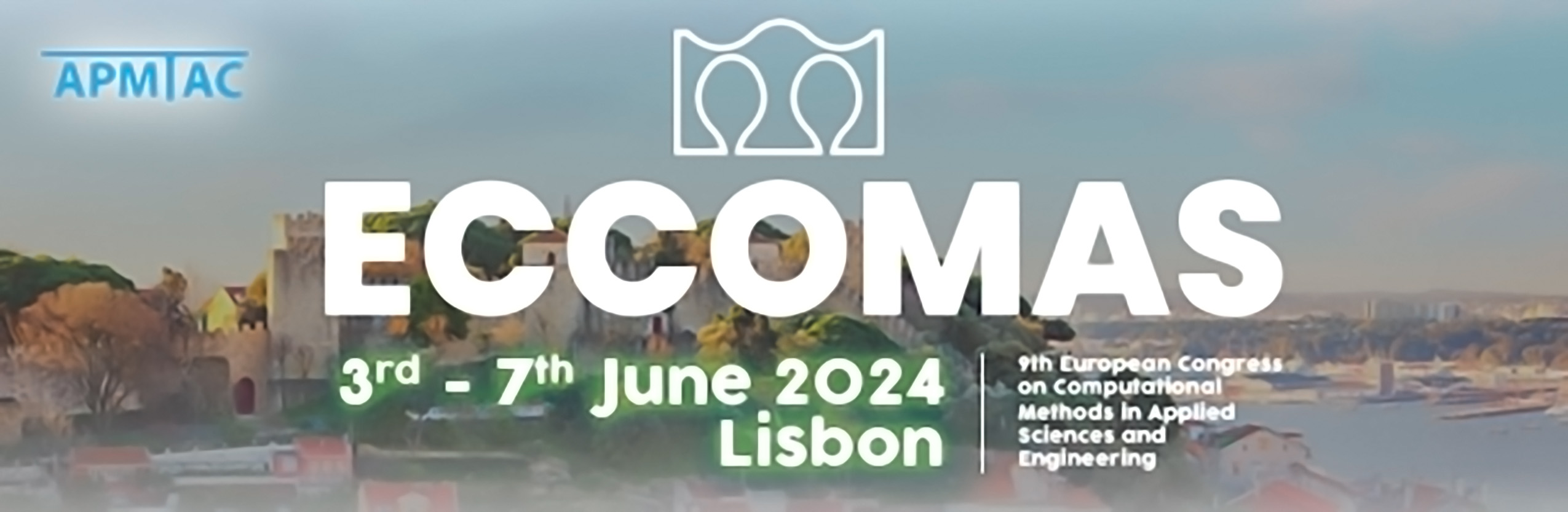
Robust Variational Physics-Informed Neural Networks
Please login to view abstract download link
We introduce a Robust version of the Variational Physics-Informed Neural Networks method (RVPINNs). As in VPINNs, we de ne the quadratic loss functional in terms of a Petrov-Galerkin-type variational formulation of the PDE problem. The trial space is a deep neural network manifold, while the test space is a nite-dimensional vector space. Whereas the VPINN's loss depends upon the selected basis functions of a given test space, herein, we minimize a loss based on the discrete dual norm of the residual. The main advantage of this approach is that the loss provides a reliable and efficient estimator of the true error in the energy norm. We test the performance and robustness of our algorithm in several steady-state advection-diffusion problems in one and two dimensions. The numerical results perfectly align with our theoretical findings, showing that our estimates are sharp.