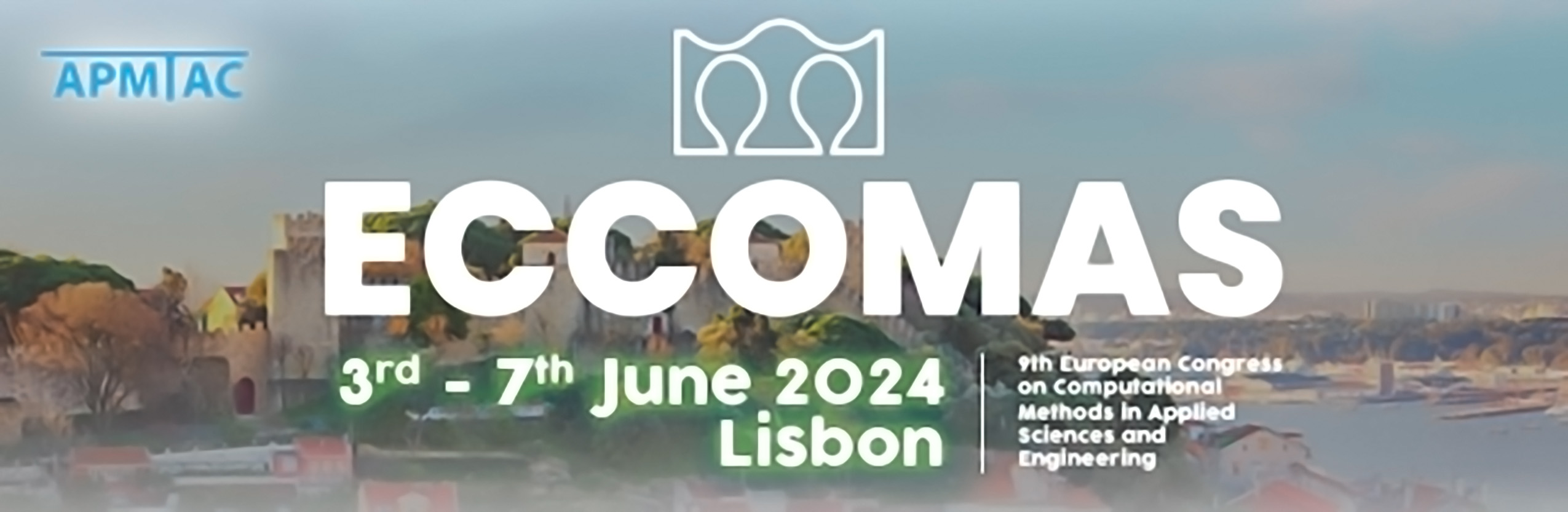
Implementing a Dual-Phase Machine Learning Strategy to Develop ANN-Based Constitutive Models
Please login to view abstract download link
Recently, machine learning approaches have emerged as promising alternative techniques to improve the efficiency and reliability of materials modeling approaches [1],[2] and [3]. This study presents a methodology for replacing the traditional constitutive model with a neural network-based constitutive model, constructed using data obtained from structural test. This approach initiates with an advanced inverse analysis technique to determine the parameters of a constitutive model that accurately matches experimental data. This process is conducted using newly developed SmartFIT toolbox, which implements finite element analysis, machine learning, and numerical optimization algorithms for parameter identification. The SmartFIT toolbox was employed to optimize the five essential parameters of the Johnson-Cook isotropic hardening model using the force-displacement measurements from X80 pipeline steel test. Following this parameter identification process, the calibrated Johnson-Cook model was implemented into a finite element model to generate the synthetic data under various loading conditions. The output data characterizing the material behavior derived from FE simulations was preprocessed and subsequently stored in the database to be utilized as training data for ANN. Multiple neural network architectures and various hyperparameters were systematically tested, and the best performing configuration was chosen as the final model. Following this, an Artificial Neural Network-based Constitutive Model (ANN-CM) was then integrated into FE solver Abaqus through the user-material subroutine (UMAT) for conducting simulations. To demonstrate the efficacy of this approach, a FE analysis of the shear test was carried out employing the ANN-CM, and its outcomes were compared with those generated using the conventional constitutive model. The findings showed that the ANN-CM can successfully replace the traditional constitutive models and accurately captures material behavior in accordance with constitutive laws.