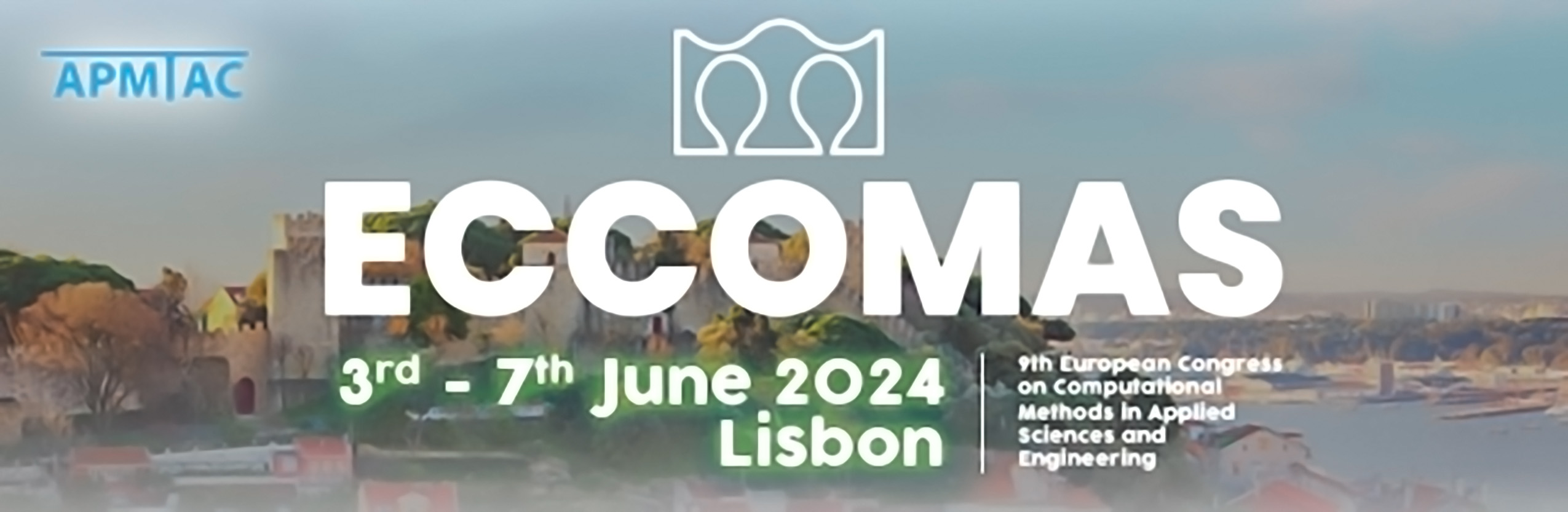
Solving partial differential equations with neural network bases
Please login to view abstract download link
Solutions of partial differential equations (PDE) are often constructed using Galerkin methods. Using neural networks in this framework is a challenge, because their internal parameters are typically found using gradient-based, iterative methods. We demonstrate that sampling a certain data-dependent probability distribution for the hidden weights and biases of neural networks allows us to construct useful neural basis functions efficiently. We then use them in a classical Galerkin ansatz to solve several PDE. Our findings are supported by numerical experiments. We will discuss competitiveness with other machine learning approaches as well as classical solvers.