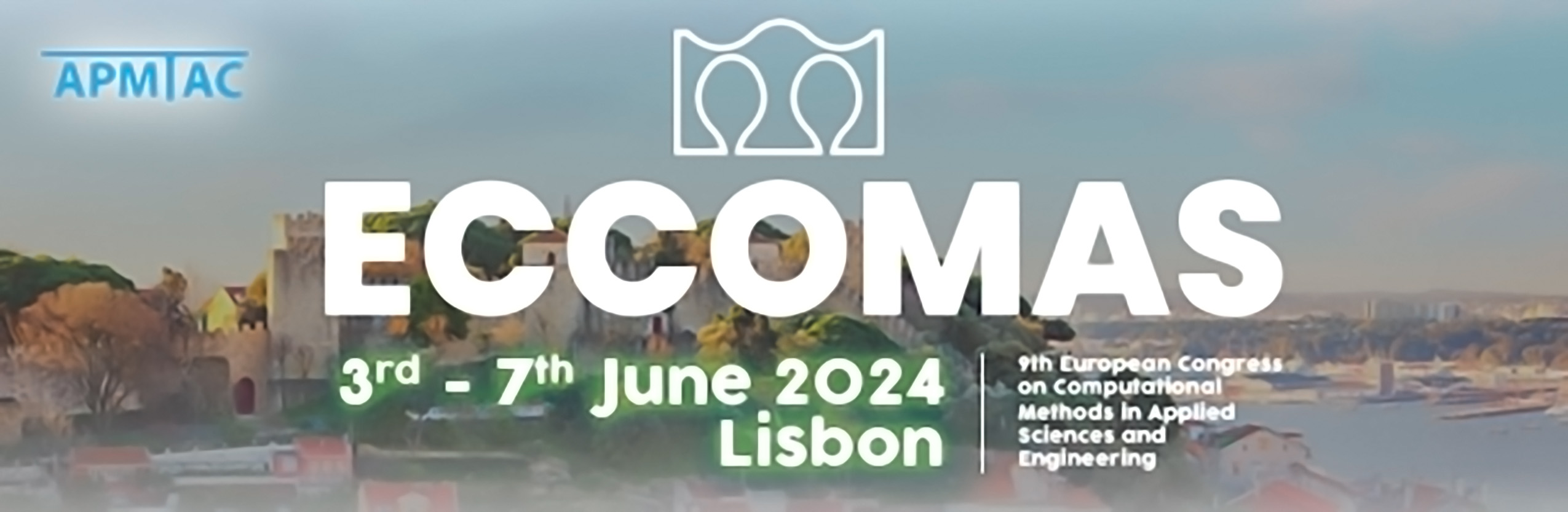
A Multi-Fidelity Surrogate Modeling Approach for Efficient Multi-Motor Loss Estimations
Please login to view abstract download link
Accurately estimating electric motor efficiency under varied operating conditions is crucial for economic operation, sustainability, and energy conservation. In industrial applications, however, we often face the challenge of predicting losses for not one but a diverse range of motors with varied configurations. In the current study, we addressed this challenge by developing a multi-fidelity Gaussian Process (MF-GP) strategy to transfer the pre-trained surrogate model seamlessly to new motors, thus mitigating the need to retrain from scratch while still preserving a high level of predictive accuracy. Our methodology was applied to estimating losses of three ABB induction motors under the inverter supply, with different rated voltage, power, and IE levels. Our results demonstrate the effectiveness of our approach for new motor model deployment, reducing time and resources for model development and training. In addition, we showed quantitatively the trade-offs between data availability and model accuracy, which provide practitioners with concrete guidelines on the minimum data requirements to achieve a desired level of accuracy.