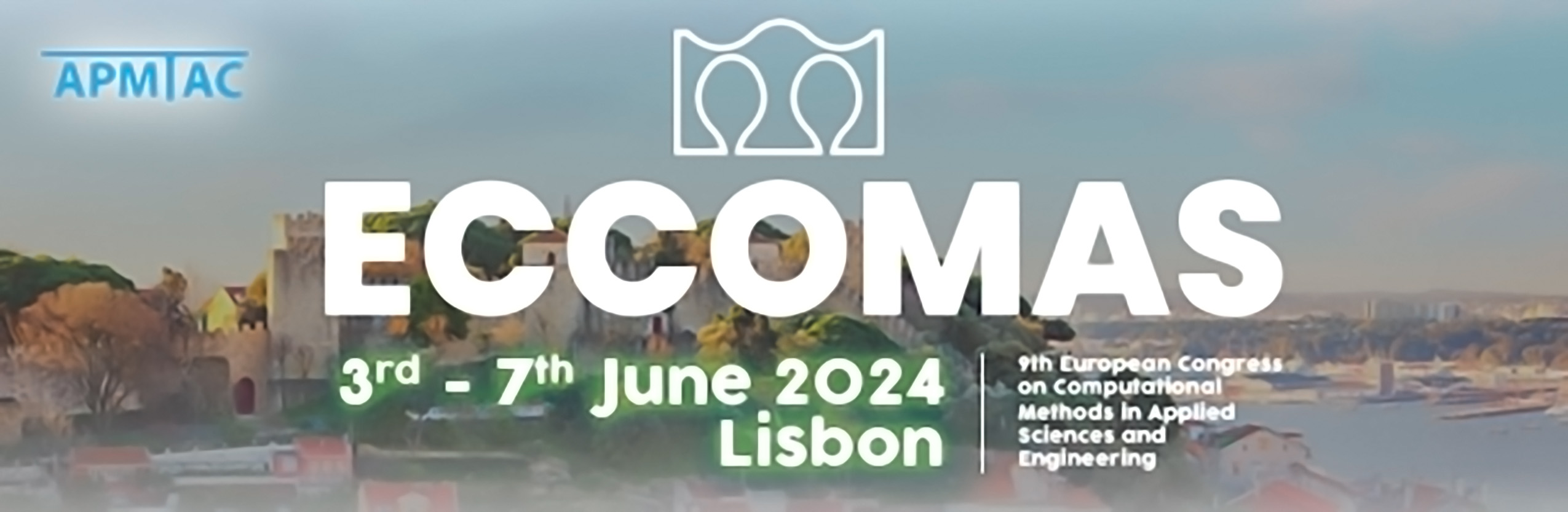
Generative strategies to empower physics-based wave propagation with deep learning. Applications to earthquake engineering and structural health monitoring.
Please login to view abstract download link
In this work, we provide a quantitative assessment of how largely can earthquake ground motion simulation and structural health monitoring benefit from deep learning generative techniques, blended with traditional numerical simulations. Two main frameworks are addressed: conditional generative approaches and neural operators. On one hand, generative adversarial learning and diffusions models are compared in a time-series super-resolution context. The main task is to improve the outcome of 3D fault-to-site earthquake numerical simulations (accurate up to 5 Hz [1,2]) at higher frequencies (5-30 Hz), by learning the low-to-high frequency mapping from seismograms recorded worldwide [3]. The generation is conditioned by the numerical simulation synthetic time-histories, in a one-to-many setup that enables site-specific probabilistic hazard assessment. On the same page, the power of Generative Adversarial Networks is successfully tackled to prove their applicability in the multi-fidelity rendering of experimental ultrasound non-destructive tests, based on the multi-modal Total Focusing Method [4], and in the prediction of the transient response of damaged shear-type multi-storey buildings [5]. In the latter case, the post-earthquake dynamic response of the multi-storey building is generated via adversarial learning technique under different damage conditions, starting from the relevant undamaged response. Finally, the successful use of neural operators to entirely replace cumbersome 3D elastic wave propagation numerical simulations is described [6], showing how this approach can pave the way to real-time large-scale digital twins of earthquake prone regions [7].