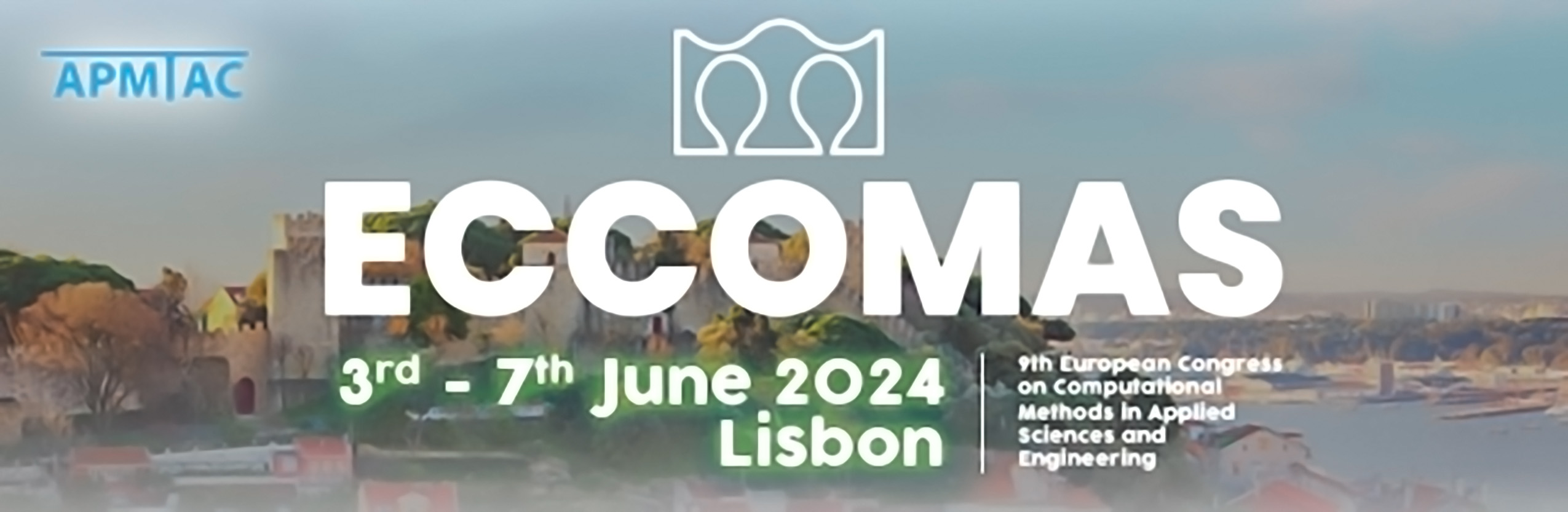
Wasserstein-VAEs for Monte Carlo ensemble generation
Please login to view abstract download link
Uncertainty Qualification in Partial Differential Equations (PDEs) tries to approximate the probability distribution of the PDE solution given the uncertainty on the data. This is typically addressed by the Monte Carlo (MC) method, which requires a large number of PDE solutions, i.e., the MC ensemble. The computational cost of building the MC ensemble can be prohibitively large for real-world applications and tools for reducing this effort are subject of intense research. A promising approach in this direction is the use of Deep Learning-based methods to approximate the MC ensemble without requiring any full model solution. Methods such as Generative Adversarial Networks (GANs) and Variational Auto Encoders (VAEs) have been proposed for these purposes. These techniques are usually based on the estimation of distances between distributions based on the Kullback-Leibler or the Jensen-Shannon divergences. Often these distances are unable to detect important differences between distributions, leading to loss of accuracy in the final approximation. To address this issue, we investigate the use in this setting of VAEs based on the Wasserstein-1 distance, which has the potential to overcome the limitations of the existing approaches. We expect the use of this distance to be especially important when dealing with stochastic PDEs whose solutions may display local features such as discontinuities or steep gradients. In particular, we employ a recently introduced dynamics-based method [2] which allows us to obtain very accurate computations of the Wasserstein distance, thus hopefully improving the resulting generator, and ultimately providing more reliable estimations of the mean and variance of the solution of parametric PDEs.