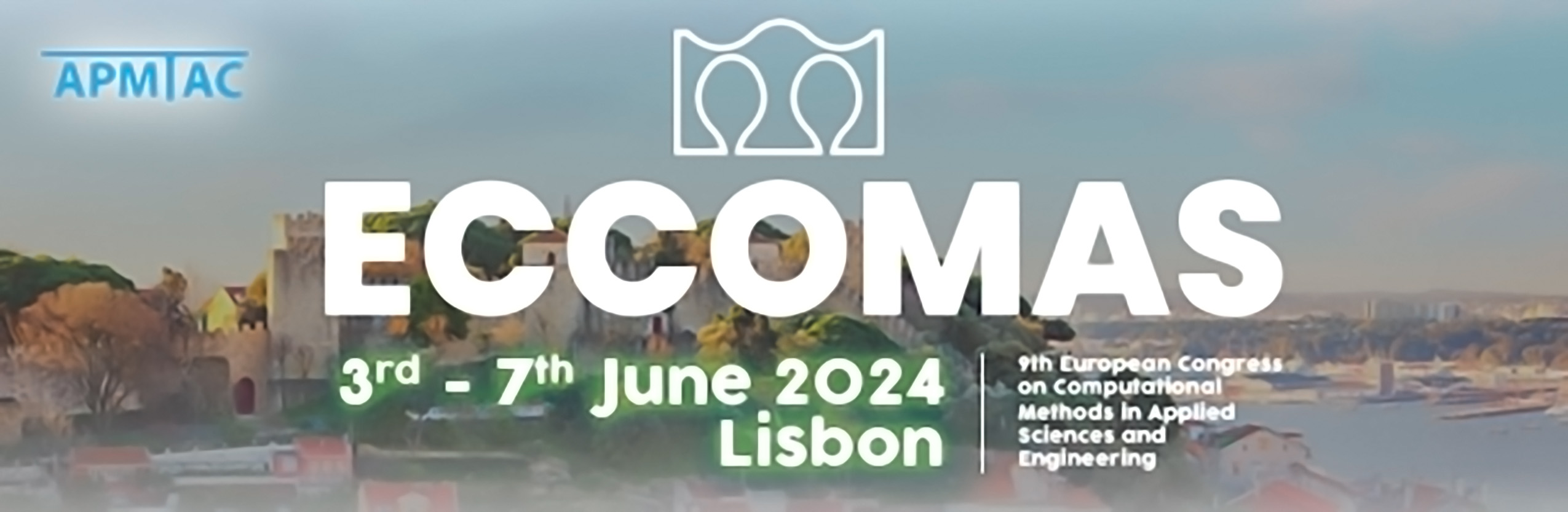
Multi-level informed robust optimization of energy systems
Please login to view abstract download link
The energy transition towards sustainable pathways demands for multi-vector interconnected models, with high temporal and spatial resolution [1]. Such models are optimized over costs in form of large Mixed-Integer Linear Programming (MILP). They further increases in complexity when considering uncertain parameters, inevitably present and expected to affect the results significantly. The state-of-the-art investigates robust, stochastic and near-optimal approaches to account for uncertainty in energy system models [2]. They rely on multiple scenarios or hypothesis-based selection, that could be computationally prohibitive or lead to overconservative and sub-optimal solutions. Two-step approaches [3] and surrogates (e.g., chaos polynomial) have proven to be a valuable trade-off between quality and resources. A tri-level approach informed by a smart meta-modeling is herein proposed. MILP is the first inner level, which solves the operational variables for a given design and parameter set. The second cycle efficiently characterizes the feasible design space as a function of parameters via Kriging surrogate, initially developed on a small subset of scenarios. Then, the meta-model actively samples additional parametric scenarios, in order to maximize its overall confidence. The third level favors cost-effective solutions that also are insensitive to parametric variability, leveraging the surrogate. Overall, this approach is free from probability distribution assumptions. It directly aims to capture key dependencies on uncertain parameters with minimal resources, thanks to dynamic hyper-sampling on a multi-level structure. Finally, it is shown to be effective on a test case model compared to conventional approaches. [1] J. Mannhardt, P. Gabrielli, and G. Sansavini, “Collaborative and selfish mitigation strategies to tackle energy scarcity: The case of the European gas crisis,” iScience, vol. 26, no. 5, p. 106750, 2023 [2] M. Karmellos, P. N. Georgiou, and G. Mavrotas, “A comparison of methods for the optimal design of Distributed Energy Systems under uncertainty,” Energy, vol. 178, pp. 318–333, 2019 [3] D. E. Majewski, M. Lampe, P. Voll, and A. Bardow, “TRusT: A Two-stage Robustness Trade-off approach for the design of decentralized energy supply systems,” Energy, vol. 118, pp. 590–599, 201