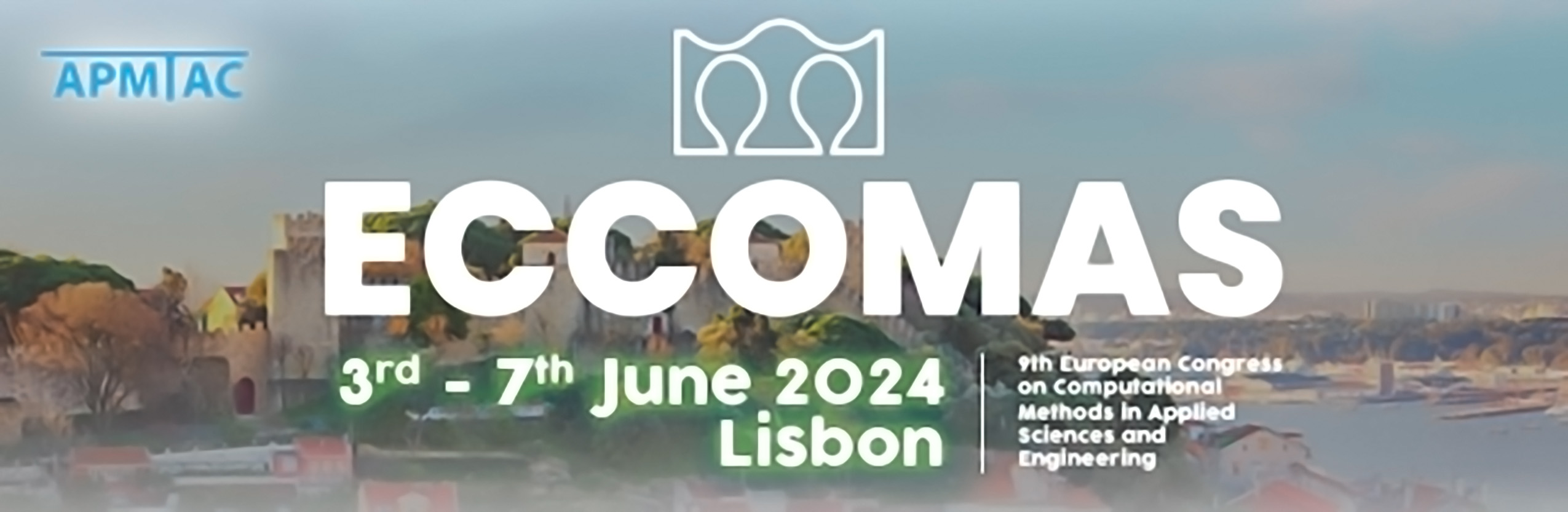
Effective application of hybrid material modeling for topology optimization of multiphase hyperelastic composites
Please login to view abstract download link
We introduce a generalized hybrid machine learning model that effectively integrates into a multiscale topology optimization framework for large deformation problems involving hyperelastic composites. With this contribution, we intend to address two key challenges: the need for an accurate and flexible constitutive model for capturing uncharacterized response of hyperelastic composites; and effective handling of material interpolation as well as excessive distortion-induced convergence difficulties in large deformation topology optimization. In contrast to traditional FE^2-type methods, we replace the computationally expensive microscale homogenization with a data-driven surrogate model. This surrogate model, developed through a principled approach grounded in hyperelastic material theory, employs a hybrid neural network architecture. Importantly, the hyperelastic surrogate model satisfies crucial constitutive conditions, including objectivity, material symmetry, symmetry of stress tensors, polyconvexity, and thermodynamic consistency. Its unique design as a strain-invariant input convex neural network and enforcement of physically meaningful restriction ensures robust predictions. To train the model, homogenized stress-strain data is obtained through numerical simulation of representative volume elements representing possible microstructures by controllably varying microscale design parameters and phase constitutive laws. An energetically motivated interpolation scheme is adopted to realize a continuous transition of microscale properties across the macroscopic optimized structure. A linearized treatment of low-density elements via stored energy density interpolation controlled by a smooth Heavyside function alleviates the non-convergence issues due to element distortion often encountered in topology optimization problems addressing large deformations. Through comprehensive validation and illustrative examples, we showcase the effectiveness of our hybrid material model-powered topology optimization framework for hyperelastic composites.