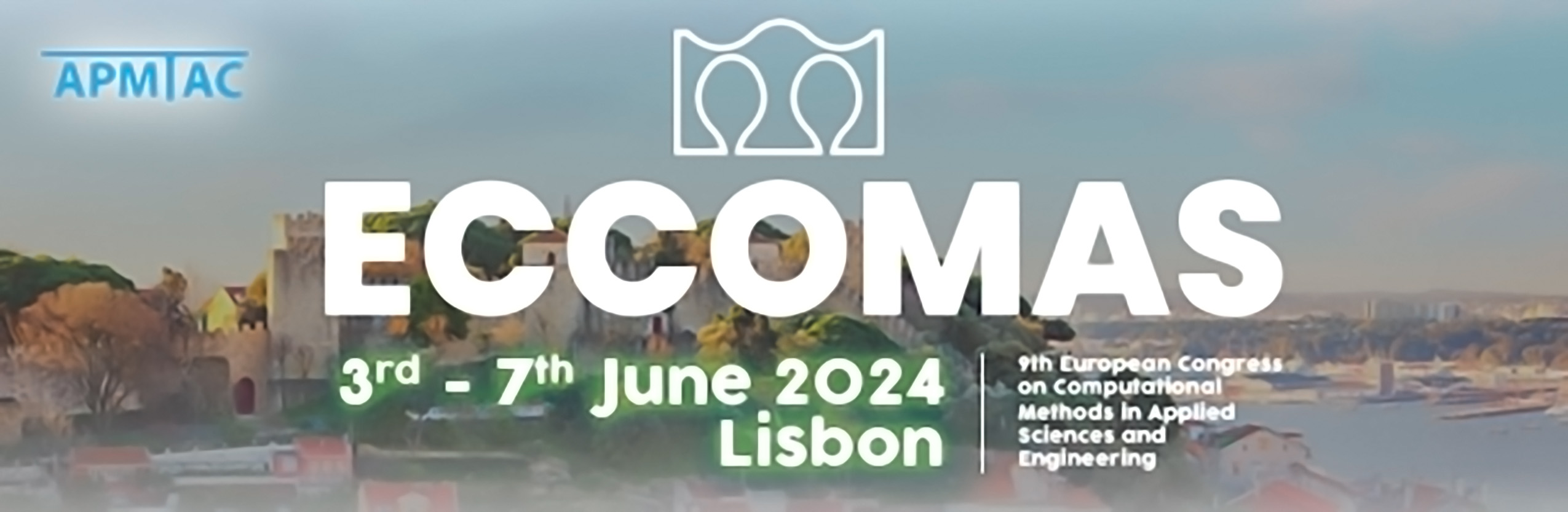
Combining Rao-Blackwellization and Importance Sampling for Connectivity Reliability Analysis of Networks with Dependent Components
Please login to view abstract download link
Modern societies operate upon various lifeline networks, including transportation, power, and gas distribution networks. Although various analytical or simulation-based methods have been developed for efficient reliability analysis of network systems, it remains challenging to handle the high-dimensional system event that arises from a large-scale infrastructure system. To address this challenge, Lee et al. [5] recently proposed a reliability method that can efficiently compute connectivity reliability of directed graphs. The method constructs a Bayesian network (BN) by using the dual graph representation of a target system. Thereby, the junction tree (JT) algorithm, a well-established BN inference method, can be applied to reliability analysis as well as complexity quantification that depends on the network topology. Building upon this previous development, in this study, we address networks described by fully correlated random variables arising from seismic uncertainties, which often cause an exponential increase in computational complexity. To this end, we propose an adaptive importance sampling technique combined with Rao-Blackwellization. By implementing the adaptive importance sampling method only for a subset of random variables in a BN and applying the JT algorithm to the remaining variables, one can efficiently combine exact analytical inference and sampling algorithm. The accuracy and efficiency of the proposed methods are demonstrated by an example of the EMA highway network with 129 edges exposed to seismic hazards.