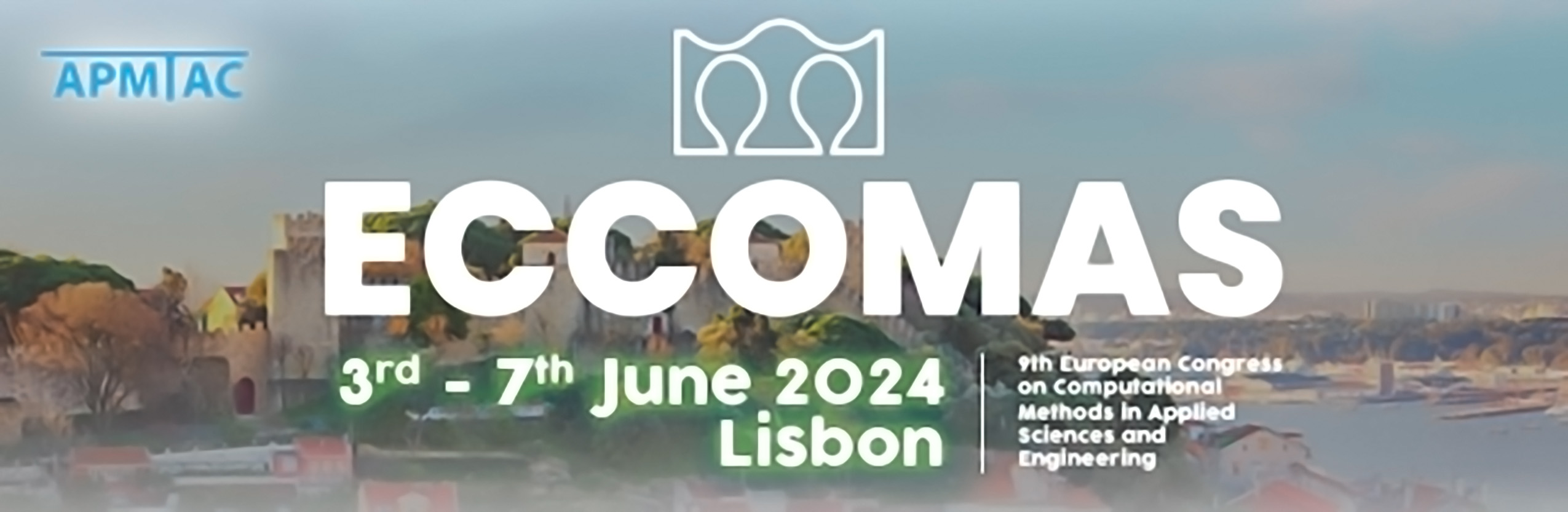
Learning Adaptive Coarse Basis Functions of FETI-DP
Please login to view abstract download link
Domain decomposition methods (DDMs) are highly scalable, iterative solvers for the solution of large systems of linear equations, e.g., arising from the discretization of PDEs. In general, the convergence rate of classical DDMs deteriorates severly for coefficient distributions with large contrasts in the coefficient function. To retain the robustness for such problems, the coarse space of the DDM can be enriched by additional coarse basis functions, often obtained by solving local generalized eigenvalue problems. This results in a computationally expensive setup of the domain decomposition preconditioner or system operator. In this talk, we train a surrogate model based on a deep feedforward regression neural network which directly learns the necessary coarse basis functions themselves. Hence, we can completely replace the computationally most expensive part of the setup, that is, the solution of local eigenvalue problems. As training data for the proposed surrogate model, we use an image representation of the underlying coefficient function such that the model can be trained independently of the underlying finite element discretization. Numerical results indicate the robustness of this approach for both, regular and irregular domain decompositions with structured and unstructured finite element meshes.