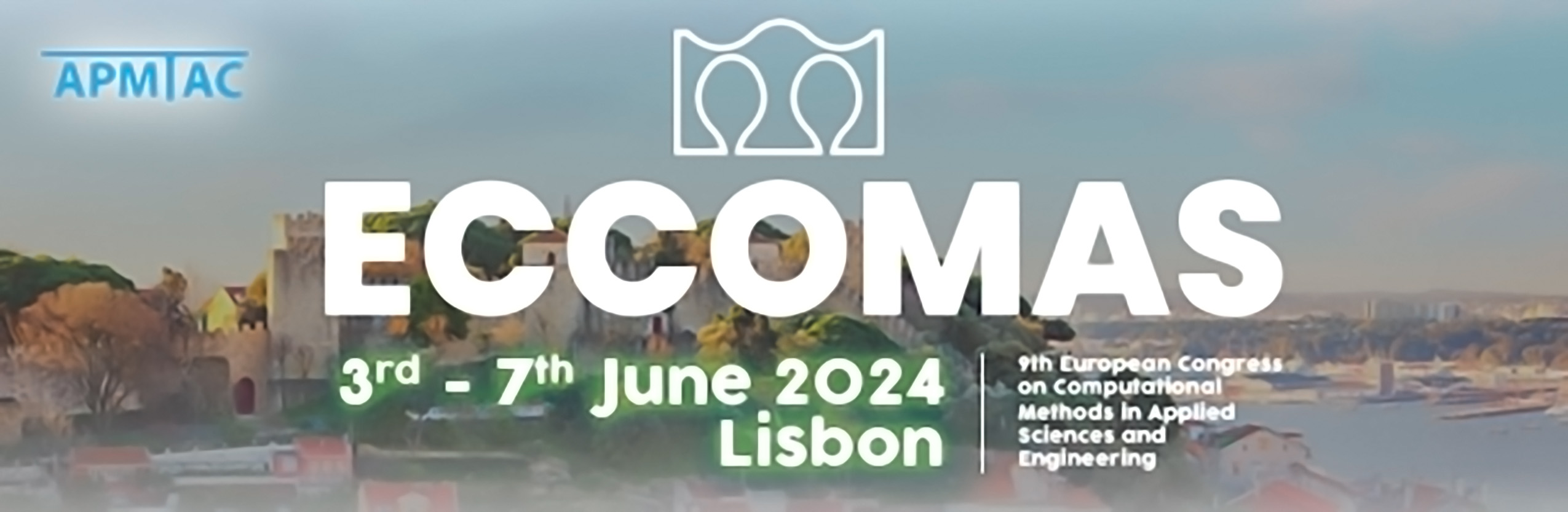
Denoising and super-resolution of 4D flow MRI using computational fluid dynamics and deep learning
Please login to view abstract download link
Introduction - 4D Flow MRI provides volumetric blood flow measurements for cardiovascular pathologies. Yet, these velocity measurements suffer from noise and limited resolution. To overcome these limitations, several studies have leveraged deep neural networks (DNNs) [1]. Training these models implies learning a mapping from noisy, low-resolution velocity fields to velocity fields that obey flow physics, thereby presenting a challenge in terms of data availability. In this study, we automate the generation of synthetic 4D flow images: from computational fluid dynamics (CFD) simulations to noisy velocity data, to obtain a large dataset that can be used to train DNNs. We then train a DNN to achieve denoising and super-resolution of 4D flow MRI in the aorta, including cases with Marfan syndrome. Methods – Starting from 126 surfaces of subject-specific thoracic aortas [2], an automatic preprocessing pipeline was developed. For each aortic surface the Laplace operator is used to obtain a point-to-point correspondence among shapes, and compute vessel centerlines. After clipping the inlet and outlets, a CFD simulation with realistic inlet boundary conditions is automatically launched. From each CFD simulation, synthetic 4D flow scans were generated using different levels of noise [1]. A 3D convolutional neural network (CNN) was trained to map noisy, low-resolution velocity images to the ground truth obtained from CFD. The CNN was trained using a patch-batch approach, and several schemes for compounding patch-level predictions were investigated. The performance of our method was assessed by computing the vector normalized mean square error (vNRMSE) between the predicted and ground truth velocity fields. Results - The implemented automatic simulation pipeline was able to generate many realistic CFD simulations in real aortic shapes. The CNN trained on this dataset was able to learn to map synthetic 4D flow data to high-resolution CFD velocity fields, obtaining, on average vNRMSE = 1.16%. Moreover, among the different prediction compounding schemes, the following vNRMSE were obtained: 1.14%, 1.18%, 1.16% for the mean, weighted mean and crop padded patch respectively. Conclusions – We implemented a method to automate CFD simulation in the thoracic aorta and generated a large dataset of synthetic 4D flow data, with different levels of noise. A 3D CNN was trained to perform denoising and super-resolution, achieving good results on a wide range on anatomies.