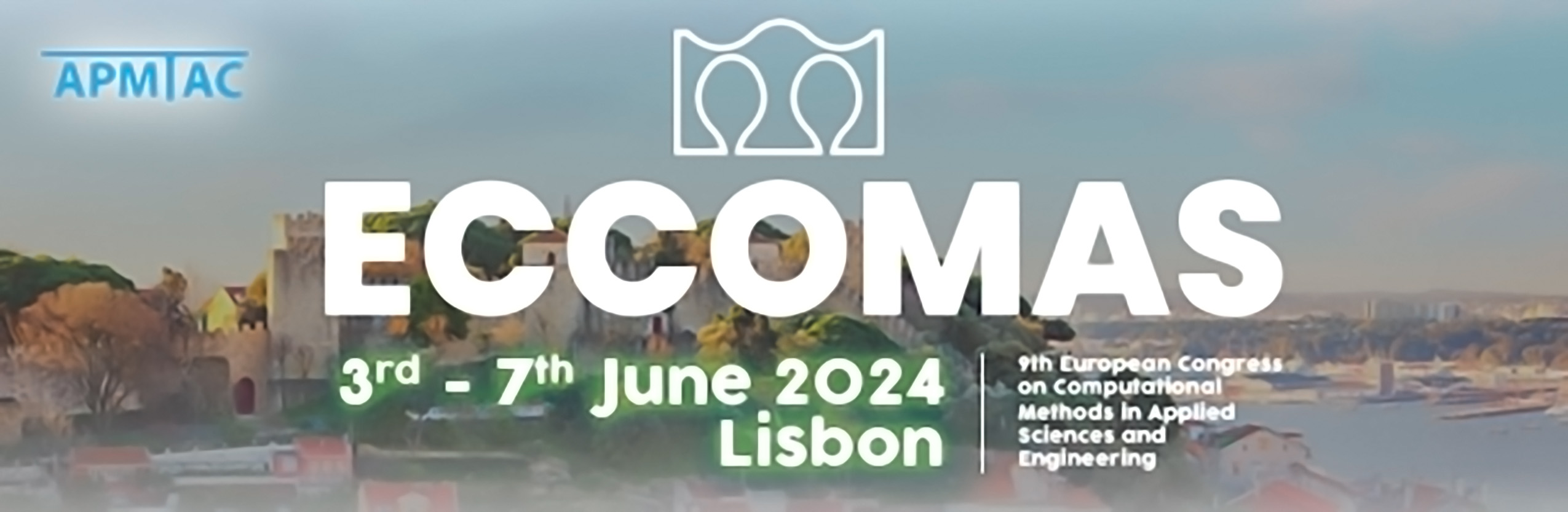
Accellerating the prediction of bone remodelling with neural networks
Please login to view abstract download link
In the field of biomechanics, the simulation of bone remodeling phenomena has been a subject of extensive research. Traditionally, these simulations have relied on numerical methods, with the finite element method (FEM) being a prominent example. While these conventional methods are known for their accuracy, they require detailed discretizations in order to accurately portray the trabecular arrangement, and due to the time dependency of the biological phenomenon, these are are iterative processes, making them highly demanding in terms of computational resources. Recently, machine learning and deep learning techniques appear as more efficient alternatives. These advanced computational approaches are gaining popularity due to their remarkable ability to yield satisfactory results in significantly less time compared to traditional methods. In this work, a neural network was trained with a set of data gathered from several finite element analyses taking in specific information about the geometry and loading conditions of the bone. Using this data, it generates a density field. With this machine learning-driven approach, the density field produced by the neural net- work closely emulates the main characteristics observed in the results from FEM analysis with an analysis time that is several orders of magnitude lower. Thus, these emerging techniques offer a promising avenue for conducting bone remodeling simulations with greater efficiency and less computational expense. REFERENCES [1] Pais, A., Alves, J. L., and Belinha, J. (2023). Predicting trabecular arrangement in the proximal femur: An artificial neural network approach for varied geometries and load cases. Journal of Biomechanics, 161, 111860.