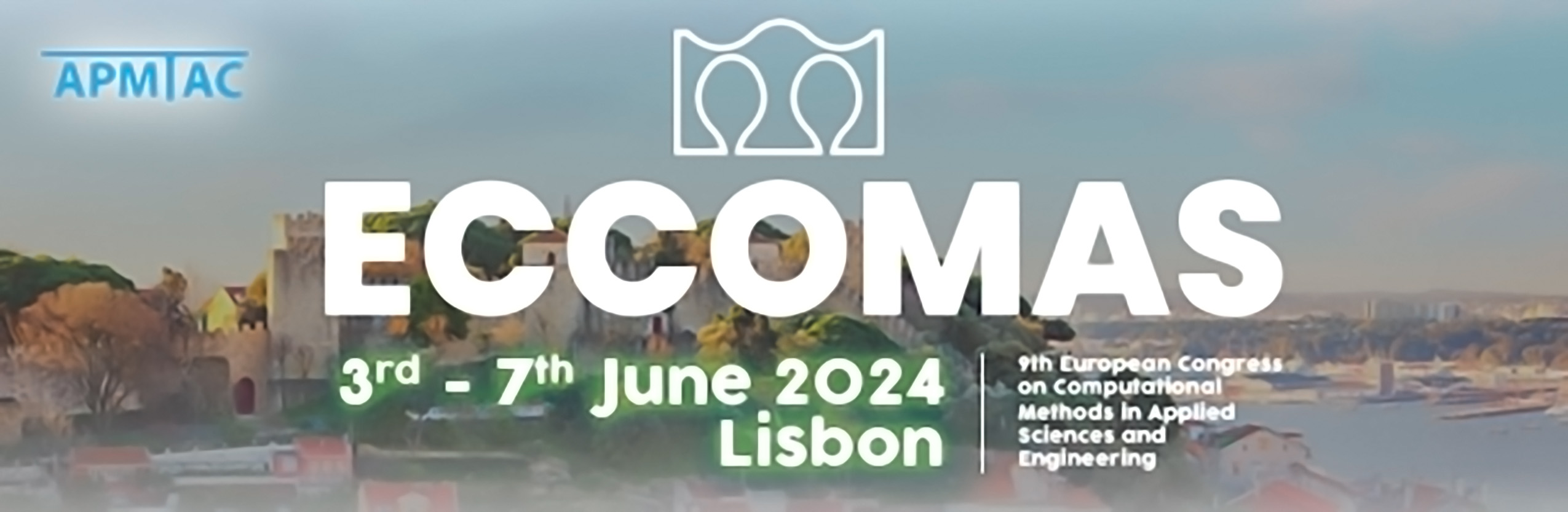
Kinetic Description and Convergence Analysis of Genetic Algorithms for Global Optimization
Please login to view abstract download link
Genetic Algorithms are a class of metaheuristic global optimization methods inspired by the process of natural selection among individuals in a population. Despite their widespread use, a comprehensive theoretical analysis of these methods remains challenging due to the complexity of the heuristic mechanisms involved. In this talk, by relying on the tools of statistical physics, we illustrate how to gain a mathematical understanding of Genetic Algorithms by showing how their behavior for a large number of individuals can be approximated through a time-discrete kinetic model. This allows us to prove the convergence of the algorithm, in particular of the individuals' mean, towards a global minimum under mild assumptions on the objective function for a popular choice of selection mechanism. Furthermore, we present a time-continuous model of Genetic Algorithm, represented by a Boltzmann-like partial differential equation, and establish relations with other kinetic and mean-field dynamics in optimization (specifically, Consensus Based Optimization and Kinetic Binary Optimization). Numerical experiments support the validity of the proposed kinetic approximation based on the propagation of chaos assumption. Computational tests investigate the asymptotic configurations of the Genetic Algorithm particle system for different selection mechanisms and benchmark problems.