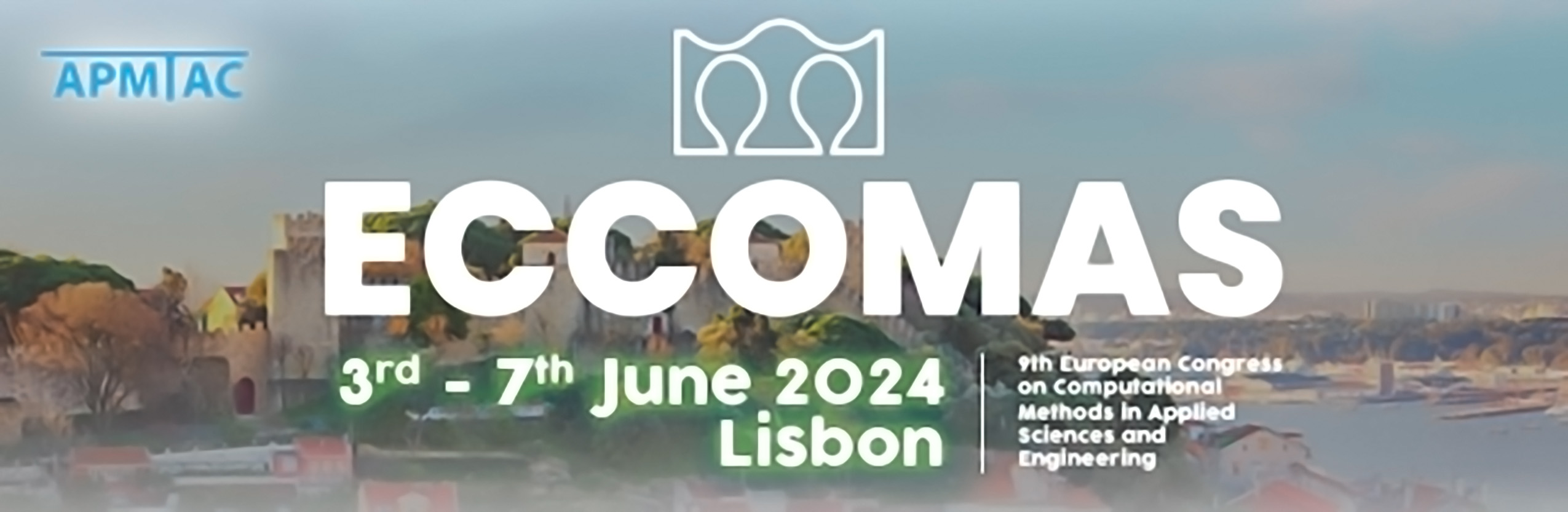
Multi-physical Modelling of Electrohydrodynamic Liquid Jets: Perspectives on Deep Learning Surrogates
Please login to view abstract download link
The dynamics of the interface of a fluid jet, when subjected to an external electrostatic field that forms a Taylor cone jet, is explored using a numerical approach based on a charge-conservative electrohydrodynamic model [1]. In our model, the simplified form of Maxwell's equations for an electrostatic field, a transport equation for electric charges, and the Navier–Stokes equations for laminar flow are solved at once. The relation between the electric field and hydrodynamic flow is coupled by the Maxwell stress tensor, which ensures the coupling of the electric surface forces to the momentum equation. A fluid volume model, using a surface compression model, offers an efficient and robust framework for modelling the behaviour of electrically driven immiscible flows. This has been demonstrated to provide accurate results, especially regarding the primary breakup of the Taylor cone jet. Full three-dimensional (3D) simulation using the continuous approach of Volume of Fluid is a complex and resource-intensive [2]. Consequently, Machine Learning techniques have been developed to create Digital Twins, enabling swift predictions across a diverse range of operating conditions with an approximate confidence ~5%. We propose the use of Artificial Neural Networks and one dimensional Convolutional Neural Networks for processing the signal of a EHD jet formed by a Taylor Cone, aiming to assess the performance of the jet, evaluating metrics like droplet size distribution, generated electric current, and droplet velocity. This allows the optimization of the input conditions to achieve the desired outcome for the application needed and a comprehensive exploration on the complex interplay of input conditions and dynamics. Our findings reveal that convolutions within layers enhance the model's learning capability, achieving a higher degree of accuracy when compared with experimental data and the scaling laws of the Taylor cone jet [1].