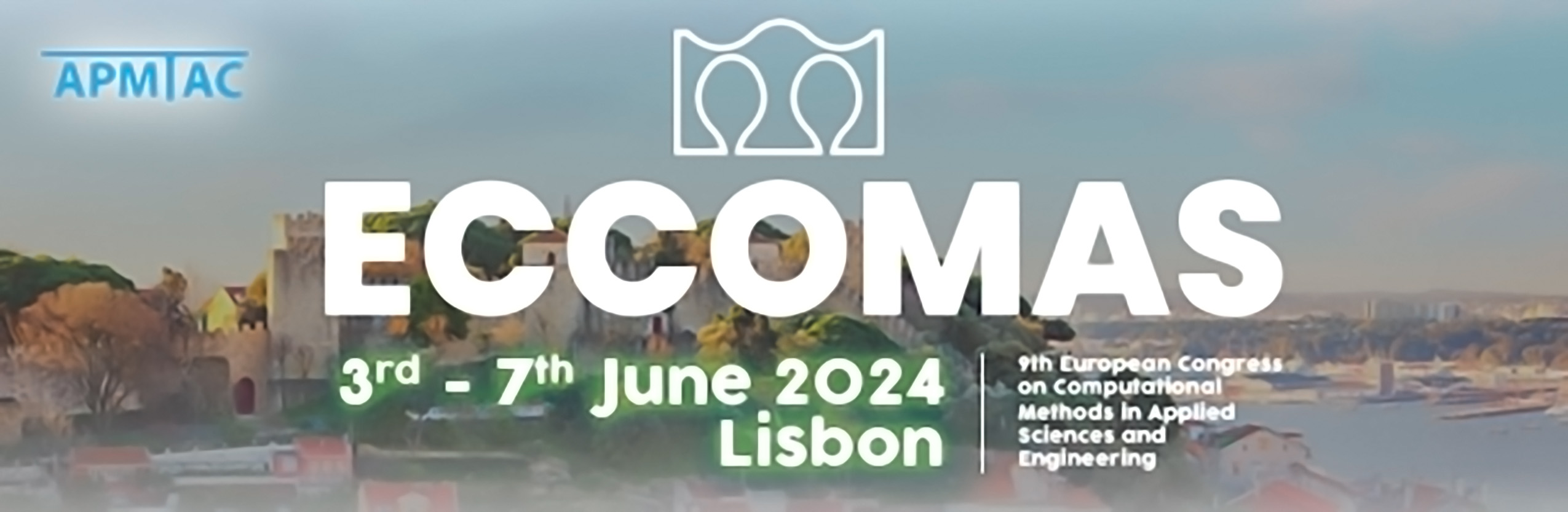
Personalized Imaging-Informed Forecasting of Prostate Cancer Progression during Active Surveillance
Please login to view abstract download link
Active surveillance (AS) is a routine clinical management option for low and intermediate-risk prostate cancer (PCa), which represent nearly 70% of newly-diagnosed cases. In AS, treatment is delayed until tumor progression towards increased clinical risk is detected via longitudinal multiparametric magnetic resonance imaging (mpMRI), serum prostate-specific antigen (PSA) tests, and biopsies. However, current AS protocols rely on an observational population-based approach, which does not account for patient-specific tumor dynamics and may result in the delayed detection of tumor progression. We propose to address these issues by leveraging personalized predictions of PCa growth and progression informed by each patient’s clinical and mpMRI data collected during AS [1,2]. Our tumor forecasts are obtained using a biomechanistic model, which describes spatiotemporal PCa growth in terms of tumor cell density as a combination of tumor cell mobility and net proliferation [1,2]. The model is defined on the patient’s prostate geometry, which is segmented on T2-weighted MRI data. Tumor cell density estimates are derived from apparent diffusion coefficient (ADC) maps obtained from diffusion-weighted MRI data. Model calibration and validation rely on assessing the mismatch between model predictions and mpMRI measurements of the tumor cell density map. We use isogeometric analysis to solve our model numerically and, hence, calculate patient-specific tumor forecasts. Additionally, we build a logistic classifier of PCa risk using model-based biomarkers calculated from the model forecasts (e.g., tumor volume, total proliferation activity) at the times of histopathological assessment (i.e., biopsy, surgery). Our results in a cohort of n = 16 PCa cases with three longitudinal mpMRI datasets show that the concordance correlation coefficient (CCC) for tumor volume is 0.89 both at calibration and prediction horizons. The corresponding spatial fits and forecasts of tumor cell density maps yielded a CCC of 0.59 and 0.60 at the second and third mpMRI scan times, respectively. Our logistic classifier of clinical risk achieved an area under the receiver operating characteristic curve of 0.90, operates at optimal sensitivity, specificity, and accuracy of 86.7%, 93.8%, and 90.3%, and anticipates PCa progression by more than 1 year with respect to standard AS. Thus, we believe that our PCa forecasting technology shows promise to guide clinical decisions for each patient during AS.