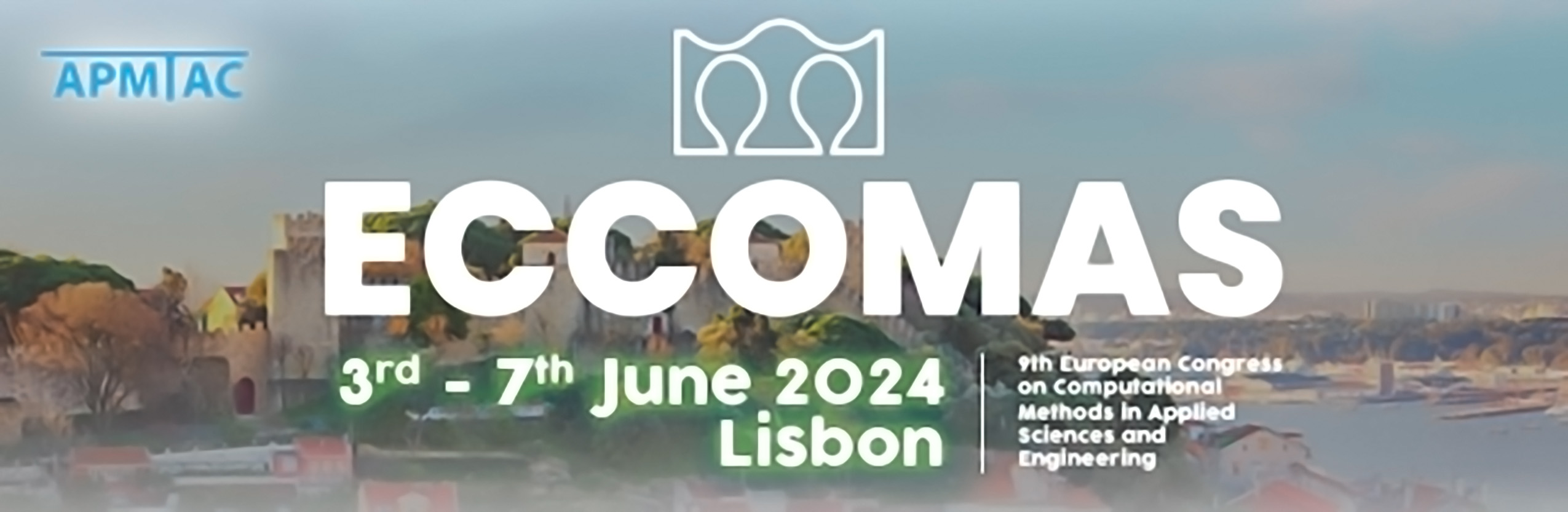
Microstructure Monitoring for Additive Manufacturing using Coupled Deep Neural Operators and Neural-Cellular-Automata
Please login to view abstract download link
The process-structure-property relationship is crucial in determining the end product's material property in the field of additive manufacturing (AM). Achieving the desired material properties and process performance requires in-depth knowledge about how process parameters affect the material properties as well as having control over tuning those parameters for obtaining desired properties. Our goal is to improve this understanding by developing novel approaches to microstructure modeling and monitoring based on data-driven approaches. This is especially important when producing functionally graded materials, where variations in microstructure can be used to create materials with spatially varying properties. The methodology utilized in our study involves the implementation of an one-way coupling approach, which incorporates two state-of-the-art data-driven methodologies: Deep neural operators (DeepONets) and neural cellular automata (NCA). The initial stage of our methodology entails the reconstruction of the full-body temperature distribution, relying exclusively on surface temperature measurements. The consistent reconstruction of the temperature field is of great importance due to the significant influence of temperature on the creation of microstructures during the manufacturing process. The implementation of DeepONet enables reliable estimation of internal temperatures, a task that was previously challenging due to limitations in direct measurement. NCA offers an advanced method to simulate the evolution of microstructures in metals during solidification processes. This novel methodology integrates the fundamental concepts of cellular automata with the computational capabilities of neural networks, particularly convolutional neural networks (CNNs). The integration approach described in this study demonstrates superior computational speed compared to standard methods, while maintaining a high level of accuracy. In summary, this study presents an innovative methodology that utilizes data-driven techniques for simulating microstructures in powder bed fusion (PBF) processes. The utilization of this technology enhances our capacity to predict and monitor the microstructure in PBF and may contribute to the fabrication of functionally graded materials.