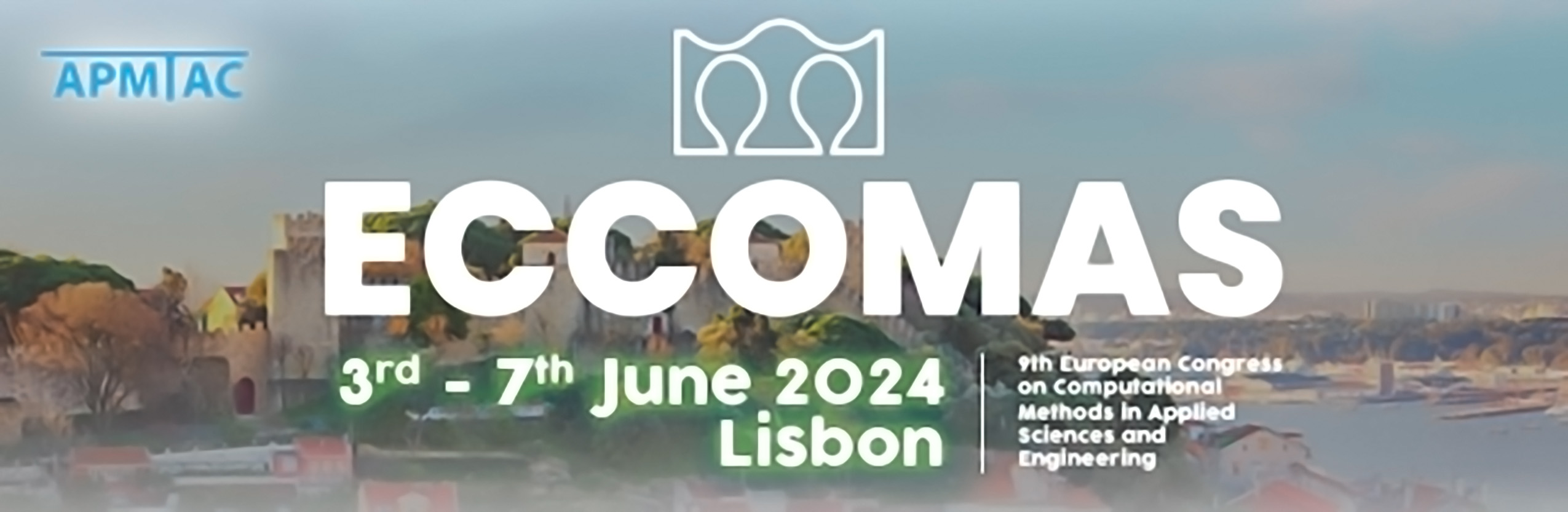
Deep Learning Surrogate Models for the Autmotive Industry
Please login to view abstract download link
When a car is exposed to lateral wind gusts, the yaw angle of the incident velocity increases, leading to an intensification of the suction at the vehicle's rear and elevated drag forces. This effect is particularly relevant in square-back vehicles, as their drag force is completely driven by the pressure on their back face. This work focuses on building a surrogate model for the changes in the aerodynamic performance with the yaw angle of a simplified square-back vehicle, the Windsor body. The training and validation datasets have been generated with wall-modeled large eddy simulations at a Reynolds number of Re = 2.9E6. The simulations have been performed using SOD2D and the obtained results are in agreement with the experimental data available in the literature. A variational autoencoder with two latent vectors has been trained with the data of the back pressure showing that the values in the latent space for the mean back pressure depend linearly on the yaw angle. Furthermore, when a set of time-correlated snapshots are passed through the encoder, the latent vector retains the dominant frequencies of the most relevant DMD modes of the system. With these properties, it has been possible to compute the mean back pressure at an unknown yaw angle with a maximum deviation of 3.1%. In the final version of this work, the extrapolation of the model outside the training range and its performance on the wake velocity will also be discussed.