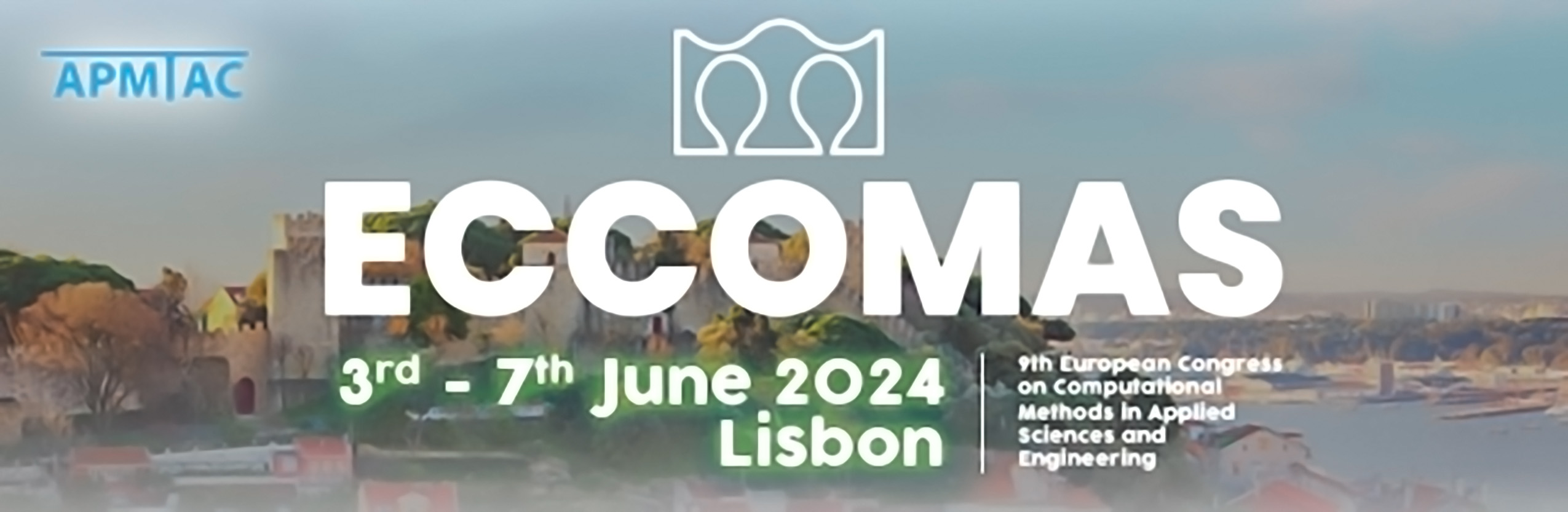
Symplectic neural networks
Please login to view abstract download link
Symplecticity refers to a geometric property that the solution of all ideal (Hamiltonian) physical systems possess, that is, the preservation of a certain 2-form. As has been repeatedly demonstrated, when learning the dynamics of such systems it is desirable to use neural models that possess this property by construction (i.e., a hard constraint). In this talk, we will leverage ideas from geometric numerical analysis to propose a novel framework for architecting and analysing symplectic neural networks. Within this framework we can describe many existing symplectic neural networks as well as propose novel ones with favourable properties (e.g., better generalisation, universality, efficient training/inference). If time permits, we will describe how symplectic neural networks can be extended to learn the dynamics of more realistic systems with forces such as dissipation.