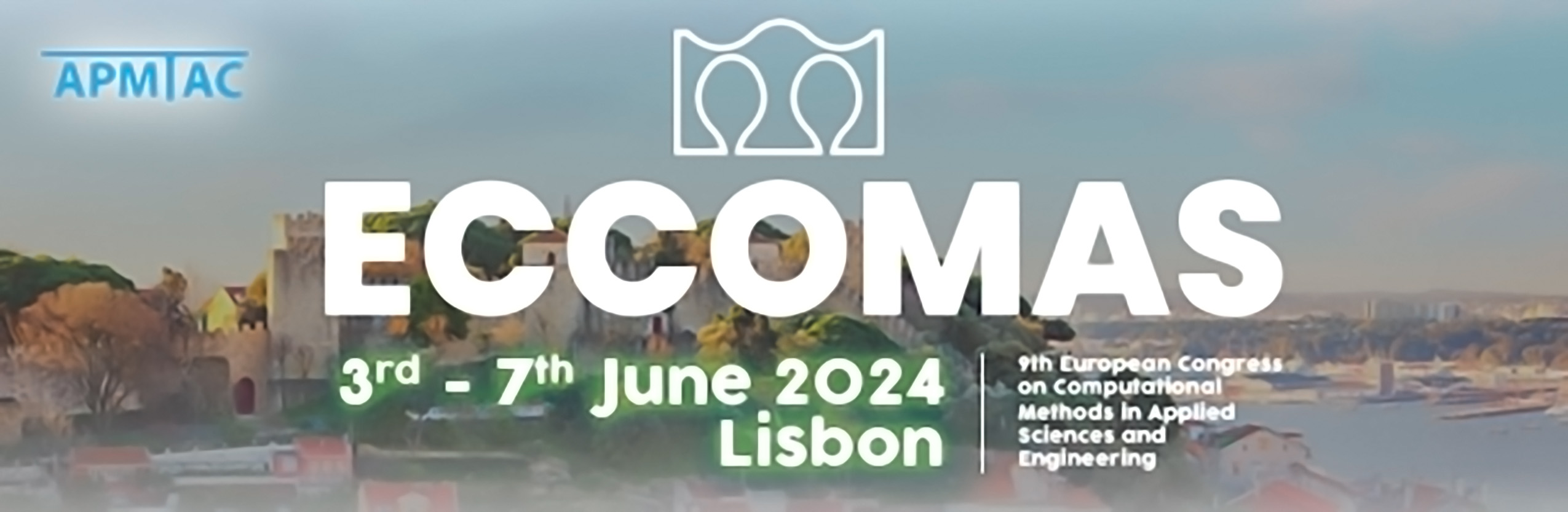
NAVEM: the Lowest-Order Neural Approximated Virtual Element Method
Please login to view abstract download link
In the last few years, Scientific Machine Learning has gained growing interest in the scientific community and, as a consequence, a plethora of new methods relying on deep neural network has emerged. In this context, we introduce the lowest-order Neural Approximated Virtual Element Method (NAVEM), a novel method to solve partial differential equations characterized by a combination of the flexibility and accuracy of the Virtual Element Method (VEM) with the efficiency and nonlinearity of neural networks. The virtual element method is a polygonal method which includes in the local space functions that are pure virtual, i.e. not known in a closed form. Thus, polynomial projectors are required to evaluate the operators involved in the equation and a stabilization term is needed to retrieve the coercivity of the method. The NAVEM main idea consists in replacing the unknown virtual basis functions with known approximations, computed through a neural network, respecting the same functional properties. This way, we are able to obtain a polygonal method that does not require any stabilization or post-processing technique. In order to stabilize the new basis functions and avoid spurious oscillations, the neural network is used to predict the function in a suitable polynomial space. Numerical tests on complex geometries are provided to validate the model and confirm its accuracy. In particular, we perform numerical experiments related to simulations in Discrete Fracture Networks, where the flexibility of polygonal methods to handle arbitrary geometries can significantly simplify the flow discretization.