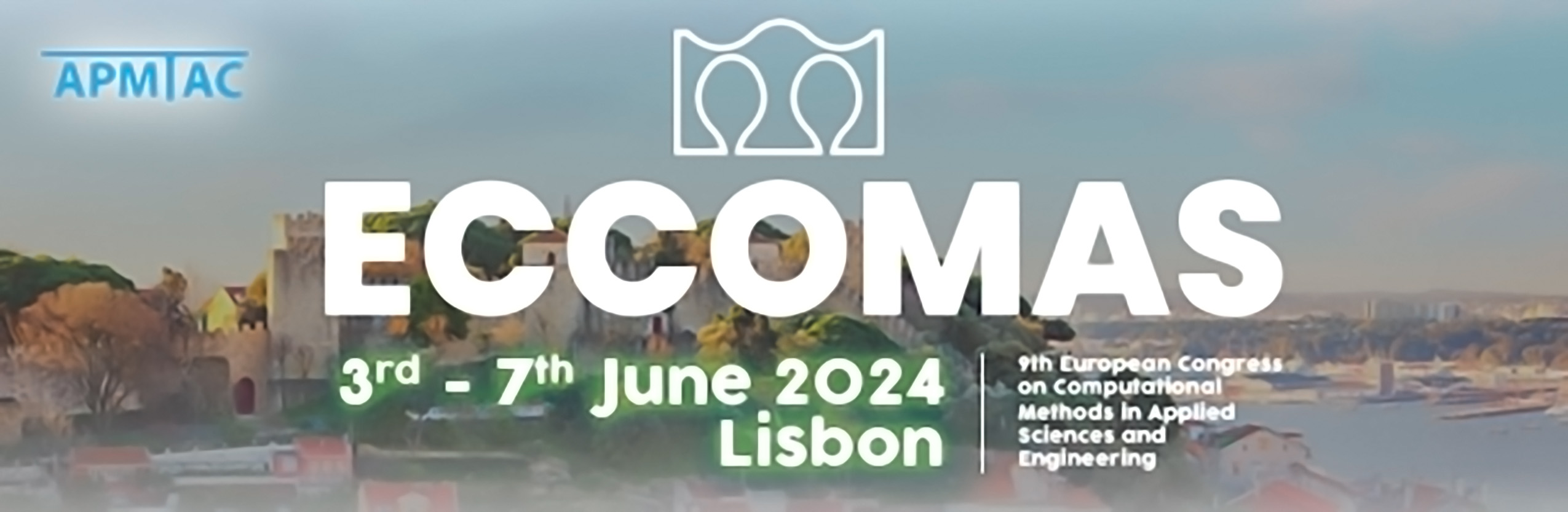
PoroTwin: Digital twins and machine learning for laboratory-scale porous media
Please login to view abstract download link
We discuss the construction of a digital twin for fluid flow and transport in laboratory porous media. The physical asset is a meter scale flow rig [1], with dynamics is monitored by imaging and potentially pressure measurements. On the digital side, forecasts of fluid transport is provided a physics-based model in the form of a PDE-based reservoir simulator. Central to the digital twin is the combination of these two data streams in real time [2]: Simulation data is accessible ahead of time, but may suffer from errors in modelling, parameters, and numerical methods. While measurements from the physical device can be considered corrections to the forecasts, they cannot be used for decision making. Our strategy to account discrepancies between simulation data and results falls under the umbrella of the Hybrid Analysis and Modeling (HAM) framwork which aims to harness the respective strength of PDE-based modelling and data-driven machine learning methods. Specifically, standard predictions from the physics-based simulator are supplemented by additional simulations enhanced to account for (unknown) errors in modelling and numerics. The enhancement is implemented by corrective source terms approximations (COSTA) [3] and computed using a convolutional neural network trained on previous laboratory experiments and simulation results. We test the application of our framework to tracer transport in porous media of varying geometric complexity. Furthermore, we discuss the extension of our framework to more complex transport processes, involving non-linear transport of multiple fluid phases, and discuss adaptations of the machine learning framework to this setting. [1] Fernø, M.A., Haugen, M., Eikehaug, K. et al. Room-Scale CO2 Injections in a Physical Reservoir Model with Faults. Transp Porous Med (2023). [2] Keilegavlen, E., Fonn, E., Johannessen, K. et al. PoroTwin: A Digital Twin for a FluidFlower Rig. Transp Porous Med (2023). [3] Blakseth, S.S., Rasheed, A., Kvamsdal, T., San, O.: Deep neural network enabled corrective source term approach to hybrid analysis and modeling. Neural Netw (2023)