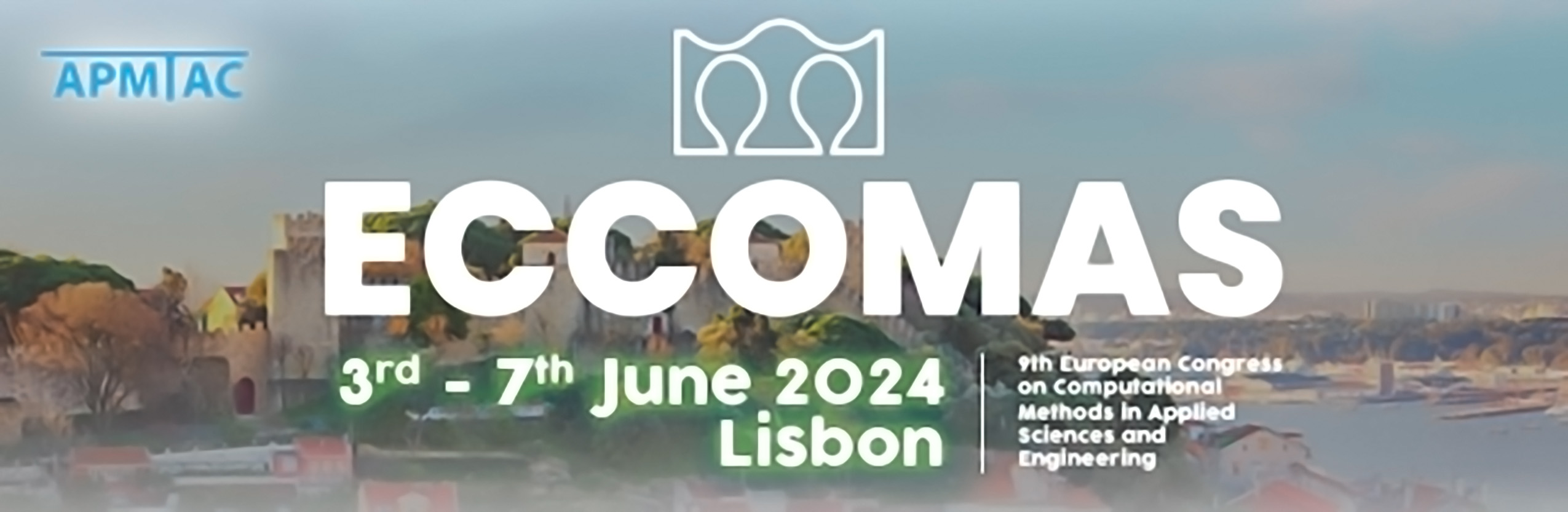
Navigating Chemical Vapour Deposition Regimes: Leveraging Unsupervised Learning for Process Insights, Surrogate Modeling, and Sensitivity Analysis
Please login to view abstract download link
The investigation of chemical vapor deposition (CVD) processes through combined experimental and computational mechanistic approaches is important for a comprehensive understanding, despite being time-consuming and resource-intensive. Particularly challenging is the identification of process conditions corresponding to distinct regimes within the Arrhenius plot, which signify the relative importance of the chemical reaction rates and the species diffusion rates in determining the deposition thickness. In this work, we present a data-driven approach for the CVD of Fe, using data from an experimentally validated computational fluid dynamics (CFD) model developed for an experimental CVD reactor: the data (thickness measurements of the coating in different locations inside the reactor) are initially compressed using principal component analysis (PCA) or even autoencoders; then hierarchical clustering, an unsupervised learning technique, arranges the data into meaningful disjoint clusters which correspond to regimes where the reaction rates either too low or where additional reactions are activated influencing the deposited film. An efficient and accurate surrogate is also developed, based on polynomial chaos expansion (PCE), and the results are assessed based on computational and experimental data retained for testing. Finally, using Sobol' indices it is possible to do sensitivity analysis to identify the influence of process conditions within each growth regime, in agreement with the mechanistic results from experimental measurements and the CFD model.