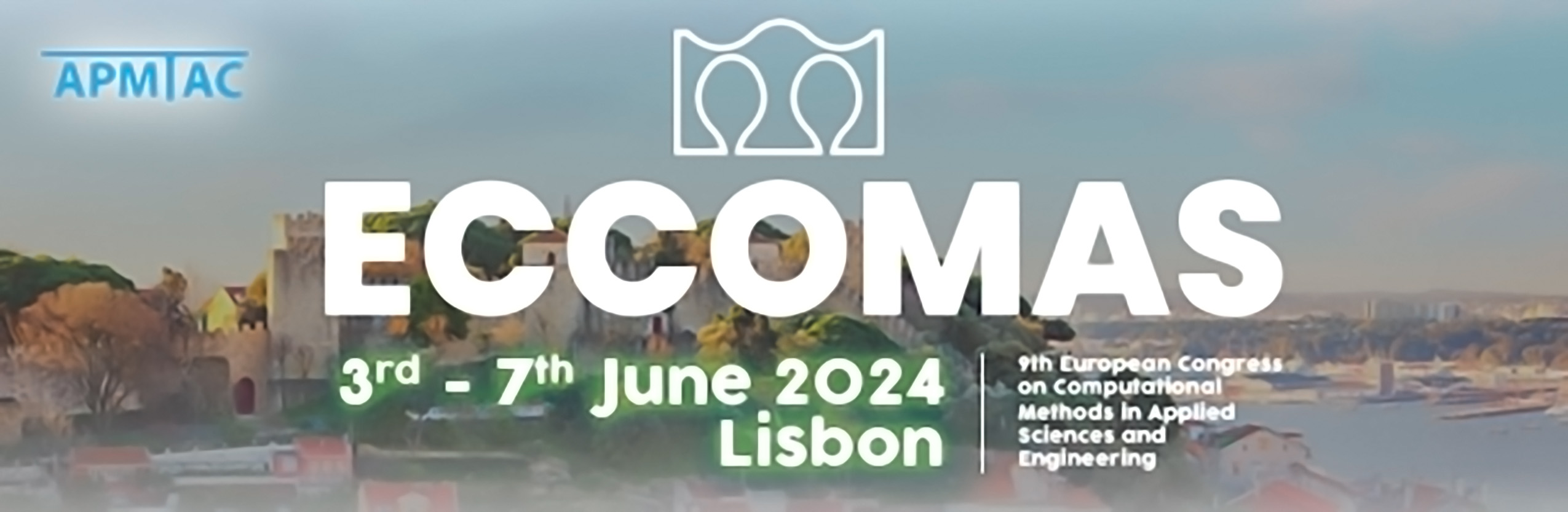
Deep Reinforcement Learning Applied to a Navigation Task in a Representative Flow Field for Urban Environments
Please login to view abstract download link
The increasing number of Unmanned Aerial Vehicles (UAV) in urban environments requires a strategy to minimize their environmental impact, both in terms of carbon footprint and noise reduction. In order to minimize these concerns, use of high-performance accelerated AI methodologies that empower innovative prediction models and optimization of flight planning with deep reinforcement learning (DRL) is needed. The european project REFMAP aims to develop a comprehensive framework in which the key aspects of sustainable aviation are taken into account, from optimal path planning to noise reduction and development of new fuels for sustainable aviation. Our goal is to develop DRL algorithms which are able to make the UAVs navigate autonomously in urban envronments, taking into account the presence of buildings and other UAVs, optimizing the trajectories in order to reduce both emissions and noise. This will be achieved using CFD simulations which represent the environment in which UAVs navigate and training the UAV as an agent interacting with a urban environment and its complexities. In this work, we show the application of DRL to planes extracted from CFD simulations of a urban environment represented by a two-dimensional flow field with the presence of two-dimensional obstacles, representing ideally buildings which are present in the path of the UAV. The presented metodology was validated by reproducing a simple but fundamental problem in navigation, namely the Zermelo's problem [1] which deals with a vessel navigating in a turbulent flow, travelling from a starting to a target point optimizing the trajectory. This is the first step towards DRL strategies which will guide UAVs in a three-dimensional flow field using real-time signals, making the navigation efficient not only in terms of flight time and avoiding damages to the vehicle, but also reducing the noise produced which would increase the level of acoustic pollution in cities. Funded by the European Union. Grant Agreement EU Horizon N.: 101096698 [1]Biferale, L. and Bonaccorso, F. and Buzzicotti, M. and Clark Di Leoni, P. and Gustavsson, K., Zermelo’s problem: Optimal point-to-point navigation in 2D turbulent flows using reinforcement learning, Chaos: An Interdisciplinary Journal of Nonlinear Science, AIP Publishing, 2019