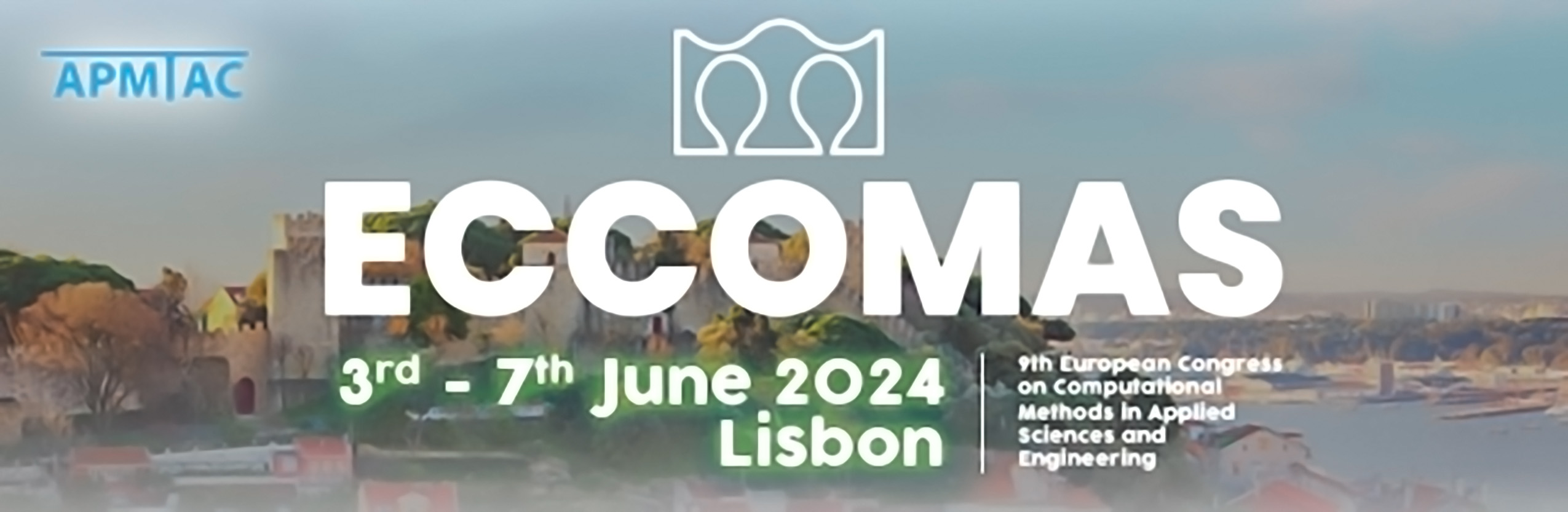
Predictive digital twins of engineering structures using neural networks and probabilistic graphical models
Please login to view abstract download link
A digital twin is a personalized virtual representation of a unique physical asset that evolves over time. It relies on a set of computational models dynamically updated to persistently mirror the physical counterpart throughout its life cycle, enabling informed decisions that realize value. Adopting a digital twin perspective for structural systems is crucial for enabling condition-based or predictive maintenance practices. Indeed, having an up-to-date digital replica of the specific structural system can result in reduced costs, increased system safety, and increased system availability. This work presents a predictive digital twin approach for the health monitoring, maintenance, and management planning of engineering structures. The asset-twin dynamical system and its temporal evolution are modeled using a dynamic Bayesian network, governing the observations-to-decisions flow and quantifying associated uncertainties. Observational data are assimilated for real-time structural health diagnostics using deep learning models. The digital state describing the structural health of the physical asset is continuously updated through sequential Bayesian inference, enabling the forecasting of future damage growth and guiding optimal actions. Computational efficiency is ensured through an offline phase, involving the generation of training datasets through reduced-order numerical models, the training of deep learning models, and the computation of a health-dependent control policy. Simulation results on a cantilever beam and a railway bridge demonstrate the capability to accurately track structural health parameters and suggest optimal control inputs. REFERENCES [1] M. Torzoni, M.Tezzele, S. Mariani, A. Manzoni, and K. E. Willcox, A digital twin framework for civil engineering structures. Computer Methods in Applied Mechanics and Engineering, Vol. 418, p. 116584, 2024. [2] M. G. Kapteyn, J. V. Pretorius, and K. E. Willcox, A probabilis- tic graphical model foundation for enabling predictive digital twins at scale. Nature Computational Science, Vol. 1, No. 5, pp. 337–347, 2021.