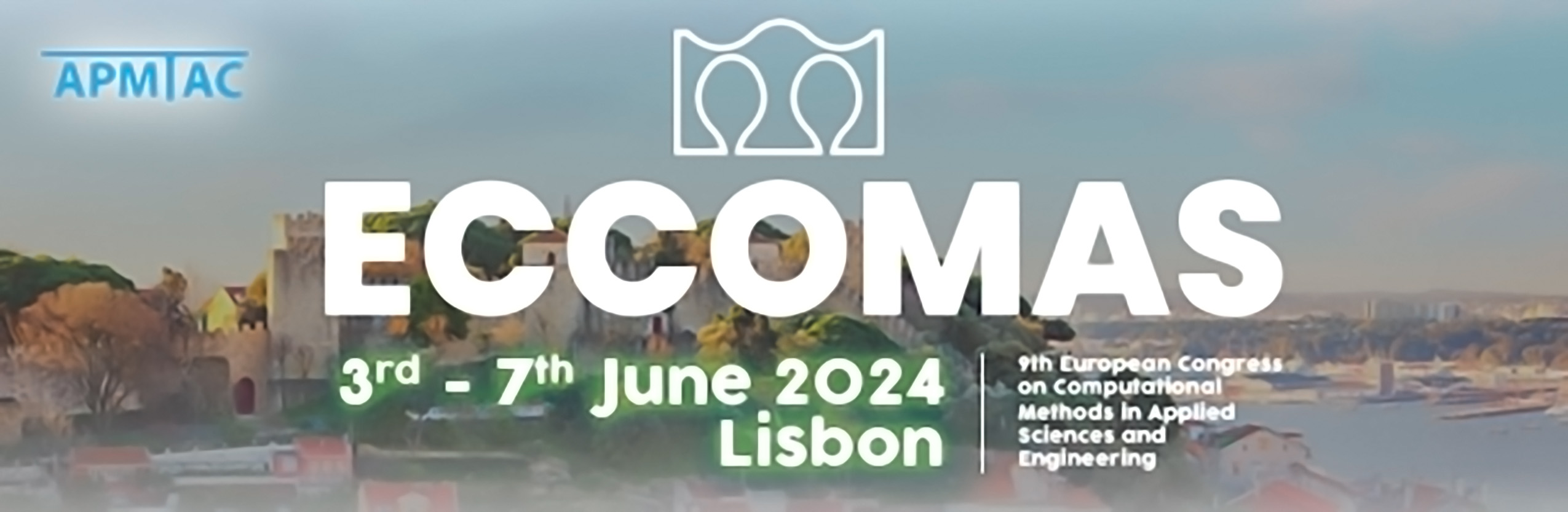
Machine-learning superpixels for 3D multiscale modelling of liver diseases
Please login to view abstract download link
Digital imaging and image analysis are rapidly developing key methods in systems biology and medicine. In order to harness the information in biological and clinical image data, robust and flexible methods are needed. During the last decade, deep learning in many cases has surpassed the performance of traditional image processing methods wherever large amounts of image data are available. However, when only few images are available, objects of interest are rare, or qualified annotations are expensive to produce, the applicability of deep learning is still limited. In such cases, interactive segmentation is a valuable tool for rapid, adaptable image analysis. We present a novel approach that combines machine learning based interactive image segmentation using supervoxels with a clustering method for the automated identification of similarly coloured images, enabling a guided reuse of interactively trained classifiers. We then use the information extracted from biological and clinical images to construct three-dimensional spatio-temporal multiscale tissue models of the human liver. The biomechanical individual-cell-based model simulations are accelerated by an experimental deep-learning approach. The constructed liver models are used to study liver regeneration after intoxication and surgery, and widespread liver diseases such as fatty liver (MASLD and MASH) and liver cancer (HCC). As a result, we have developed the first 3D human liver model of the entire disease process from a healthy liver to MASLD to MASH to HCC (Fig). We aim for a better understanding of these pathologies by inverse problem solution and a clinical translation of model-based improvements of therapy.