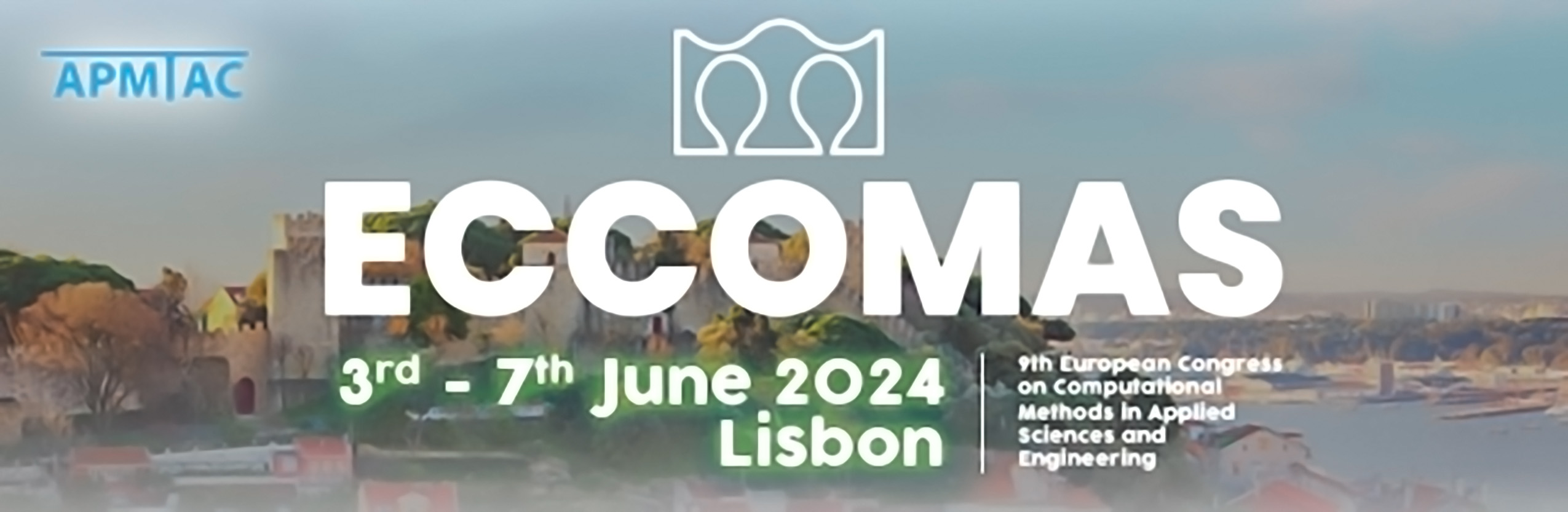
Data Science Approach to Modeling Data Center Energy Efficiency
Please login to view abstract download link
The rapid growth of digitization has led to a skyrocketing increase in data centers' energy consumption. By 2030, they are expected to consume 13%, potentially up to 21% of global energy demand [1]. This could lead to significant operational costs, power security impacts in the energy ecosystem, and environmental threats. Integrating the IoT, sensors, and intelligent devices has significantly contributed to generating vast operational management data from various aspects of the data centre industry. Effectively modelling and processing this data could improve energy efficiency, ensure reliability, reduce operating costs, and sustainably manage data centres. However, prior heuristics, statistical, and engineering methods could not be effective for modeling and simulating this data. This paper develops a data-driven AI-based framework for modeling data center efficiency that learns from actual operational data. The framework leverages several machine-learning algorithms. Specifically, we proposed a hybrid deep neural network (DNN) called CNN-LSTM (Convolutional Neural Network and Long-Short-Term Memory), a predictive analytics model to predict and optimize data center energy efficiency accurately. The model extensively trained and validated using actual data from a High-Performance Computing (HPC) data center at the ENEA CRESCO cluster in Italy. 70% of the data used for training and the remaining 30% for testing. The robustness of our model is then eventually identified through a sensitivity analysis optimization strategy utilizing various cooling system fan speed-controlled variable setpoints. The optimum model is then identified when the fan speed setpoint decreases by -50% with an average error RSME of 0.0401, optimizing energy consumption by an average of 2.29 kWh. Hence, by simulating the trained model, data center operators can proactively scrutinize, plan, schedule, and optimize their data center energy efficiency while minimizing operational and environmental costs.