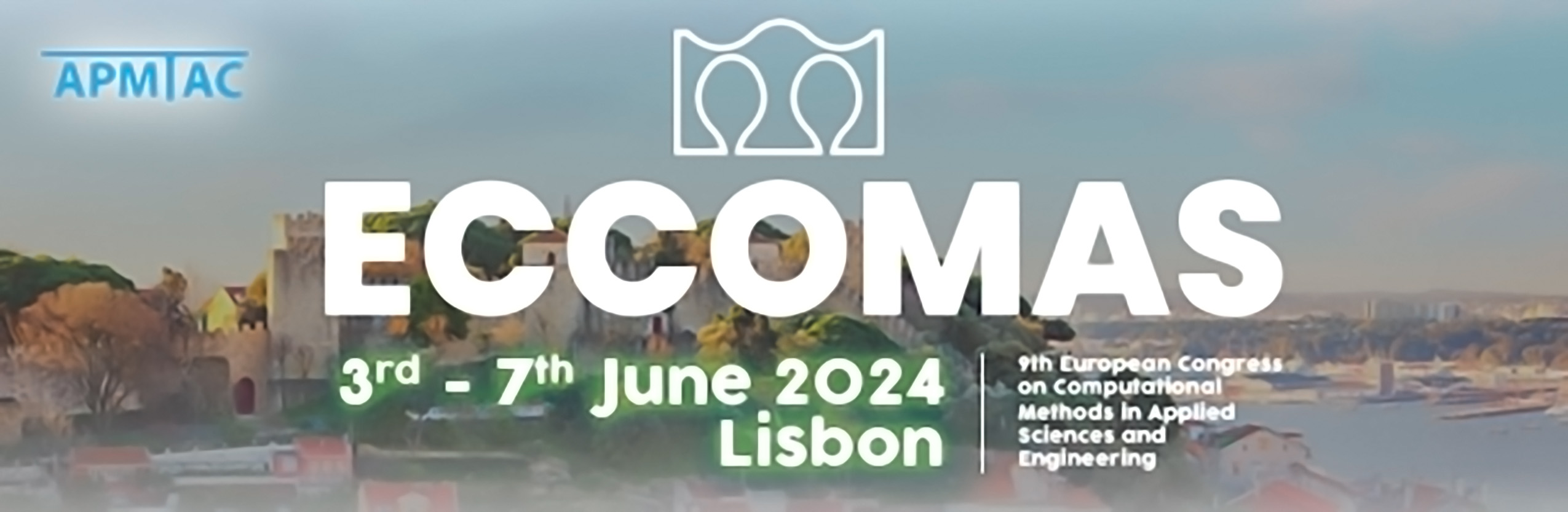
Parameterized hyperelastic material modeling and multiscale topology optimization with physics-augmented neural networks
Please login to view abstract download link
In the last decades, a vast amount of highly specialized metamaterials has been developed and, with advancing requirements in engineering applications, the trend is growing. Often comprised of complex microstructures with parametrized geometry, these materials can be tailored for each specific application. Examples include lattice-metamaterials with varying radii or fiber-reinforced elastomers where the volume fraction of the fibers might vary. Since such materials behave highly nonlinear, their mechanical description is challenging. Thus, we envision the use of physics-augmented neural networks (PANNs), circumventing the current limitations of analytically formulated multiscale material models. In [1], neural networks are applied to formulate parametrized hyperelastic constitutive models. The models fulfill all common mechanical conditions of hyperelasticity by construction. In particular, partially input convex neural network (pICNN) architectures are applied based on feed-forward neural networks. Receiving two different sets of input arguments, pICNNs are convex in one of them, while for the other, they represent arbitrary relationships, which are not necessarily convex. In this way, the model can fulfill convexity conditions stemming from mechanical considerations without being too restrictive on the functional relationship in additional parameters, which may not necessarily be convex. Two different models are introduced, where one can represent arbitrary functional relationships in the additional parameters, while the other is monotonic in the additional parameters. As a first proof of concept, the model is calibrated to data generated with two differently parametrized analytical potentials, whereby three different pICNN architectures are investigated. In all cases, the proposed model shows excellent performance. Furthermore, in [2], hyperelastic PANN constitutive models are applied for topology optimization (TO). Using a level-set TO approach, the applicability of hyperelastic PANN constitutive models in 2D and 3D optimization scenarios is demonstrated. The optimized designs received with the PANN constitutive model are in excellent agreement with the ones received with their ground truth counterparts. Furthermore, a parametrized PANN from [1] is applied for multiscale TO. In the optimization process, both the geometry of the macroscale and microstructural parameters are optimized, where the TO with the PANN model again shows excellent results.