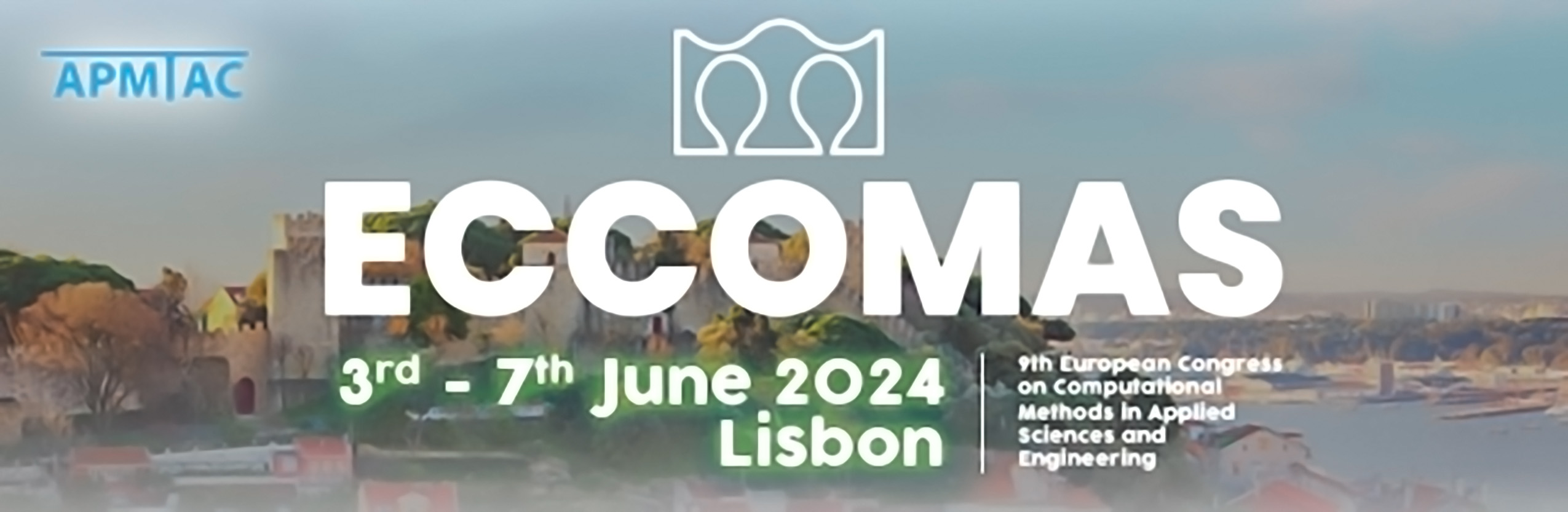
Data-Driven Acceleration of Statistical Convergence in Turbulent Flows
Please login to view abstract download link
Direct numerical simulations (DNS) provide accurate and reliable information for the physics discovery and modeling of turbulent flow phenomena, especially about their inherent multiscale nature in terms of kinematic and dynamic behavior. However, obtaining converged statistics of high-Reynolds-number turbulent flows typically requires fine-resolution DNS experiments time-integrated for very long periods, which result in significantly expensive and time-consuming computations. This work introduces an innovative data-driven methodology tailored to accelerate statistical convergence of turbulent flows. Its successful development will disruptively transform the computational study and optimization of turbulent flows by largely reducing compute times. The strategy is based on hastening the convergence of turbulent statistics by introducing controlled ``on the fly'' perturbations to the Reynolds stresses within a physics-constrained realizable framework. The method employs reinforced learning to determine the perturbations within the six degrees of freedom of the tensor, encompassing its magnitude (trace), shape (eigenvalues) and orientation (eigenvectors). The novel data-driven framework will be comprehensively described and its performance carefully assessed. The tests will consider the canonical one-dimensional turbulence (ODT) and three-dimensional turbulent channel flow problems at various Reynolds numbers, and will analyze in detail the convergence behavior and speedup factors of first- and second-order turbulent flow statistics.