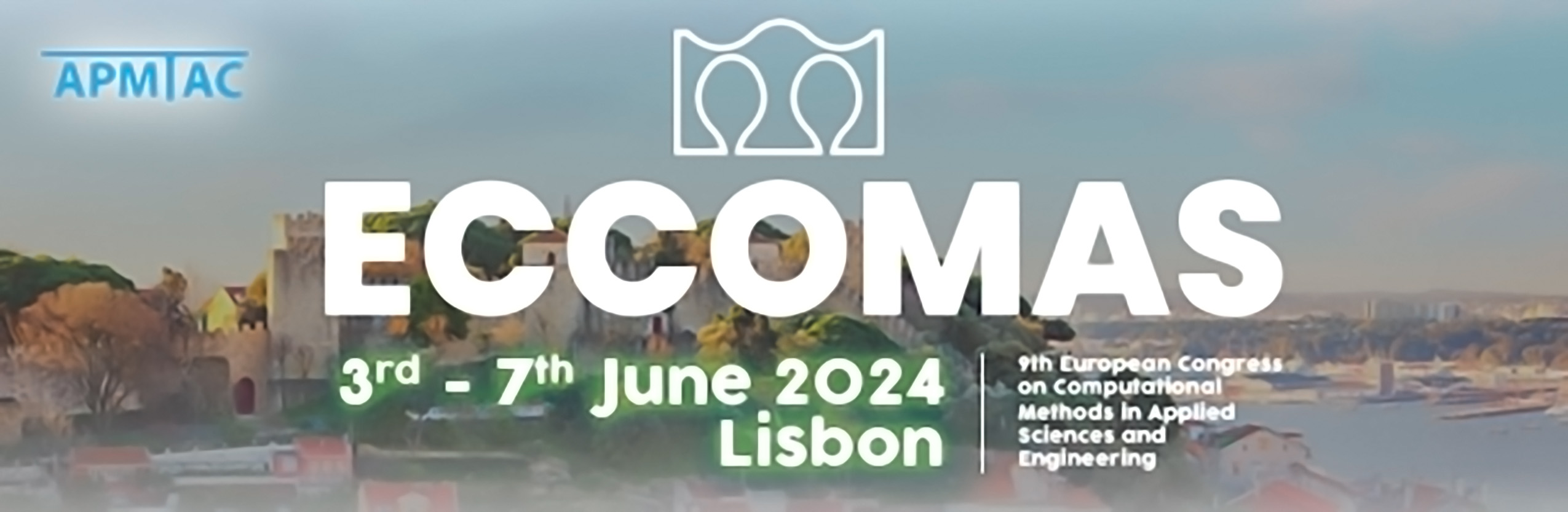
Inverse Uncertainty Quantification in Passive Automobile Safety: Bayesian Inversion for Optimal Safety Ratings with Robustness Quantification
Please login to view abstract download link
We introduce an innovative approach to inverse uncertainty quantification in the domain of passive automobile safety, utilizing Bayesian inversion techniques. Our method computes the required input distributions of key safety variables to achieve a desired distribution in the results space. This proactive methodology shifts from a traditional trial-and-error to a question-oriented approach: "What adjustments are necessary to ensure optimal occupant protection?", conceptualizing the target as a distribution rather than a fixed outcome. A central feature of our methodology is its ability to recognize alternative solutions, yielding similar results. Secondly, balancing between achieving extreme safety results and maintaining robustness under various conditions is addressed with a robustness metric to assess such solution sets. Additionally, the approach benefits from leveraging the analytical describability of certain parts of the safety engineering process. This reduces the size of the required data set and enhances the practicality of the method. Distinctively, we demonstrate our method's effectiveness on an industry problem, underscoring its scalability and real-world relevance. This demonstration confirms the feasibility of applying our approach in complex automotive safety scenarios. By integrating Bayesian inversion with a data-driven strategy and introducing a robustness quantification metric, our approach offers an efficient and versatile tool for enhancing the safety engineering process in the automotive industry.