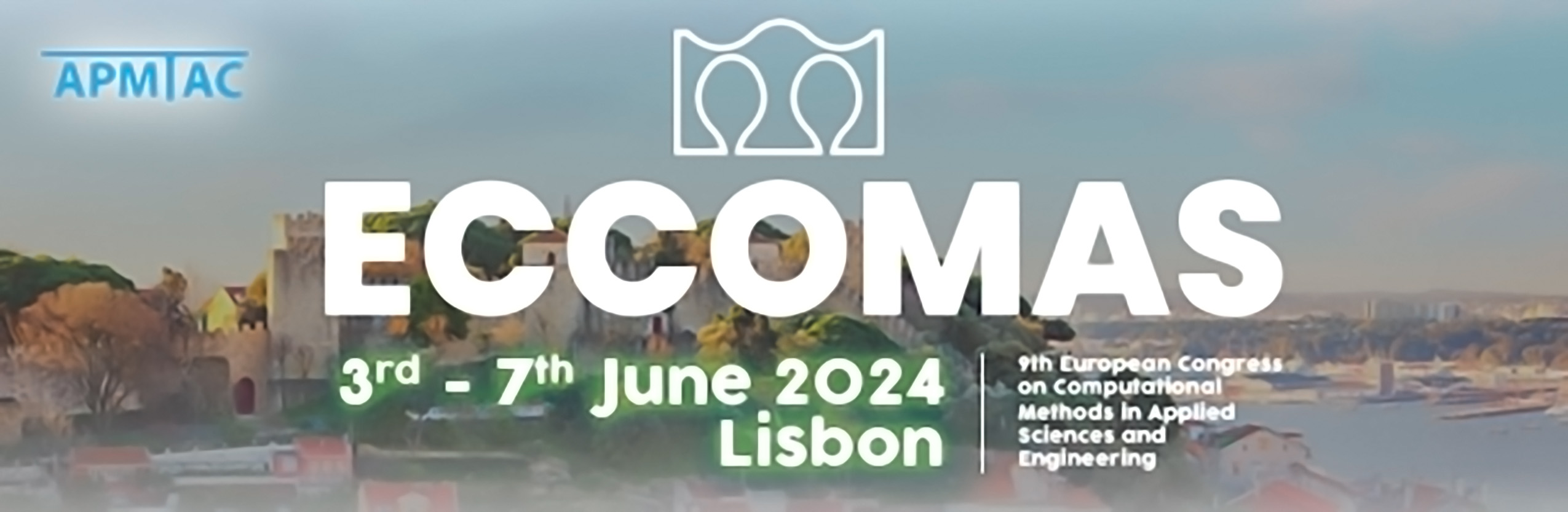
Streaming Algorithms for Estimation of Time-averaging Uncertainties in Turbulence Statistics
Please login to view abstract download link
In computational and experimental studies, the turbulence statistics computed by sample mean estimators are uncertain due to the finite number of samples used. The existing methods for estimating such uncertainties typically work in an offline mode meaning that they need to access to the full set of samples at once. This can be a problem when dealing with large-scale turbulence simulations where storing the time series data of flow quantities over a large domain of interest on disk is not a practical option. Besides this, providing the possibility of online monitoring of the convergence of statistics in turbulent flow simulations can be an extremely useful feature in practice. We present a framework recently developed for in-situ (online/streaming) estimation of uncertainty in time-average quantities of turbulent flows [1]. The workflow can be used in both non-intrusive (via an interface with the CFD software) and intrusive (implemented in the CFD software) ways. The two main novel contributions are a function to create a smooth model for the autocorrelation functions (ACF) based on a small number of sample estimated ACF values, and a low storage streaming algorithm for estimation of the sample ACFs. The uncertainties are estimated from a closed-form mathematical expression. The framework is validated for a set of wall-bounded turbulent simulations over structured meshes and when using an adaptive mesh refinement strategy. The high computational efficiency of the framework is demonstrated by performing weak- and strong-scaling tests. The methodology and workflow are general, hence they can be used for simulation of any dynamical system that produces quantities with autocorrelated time samples.