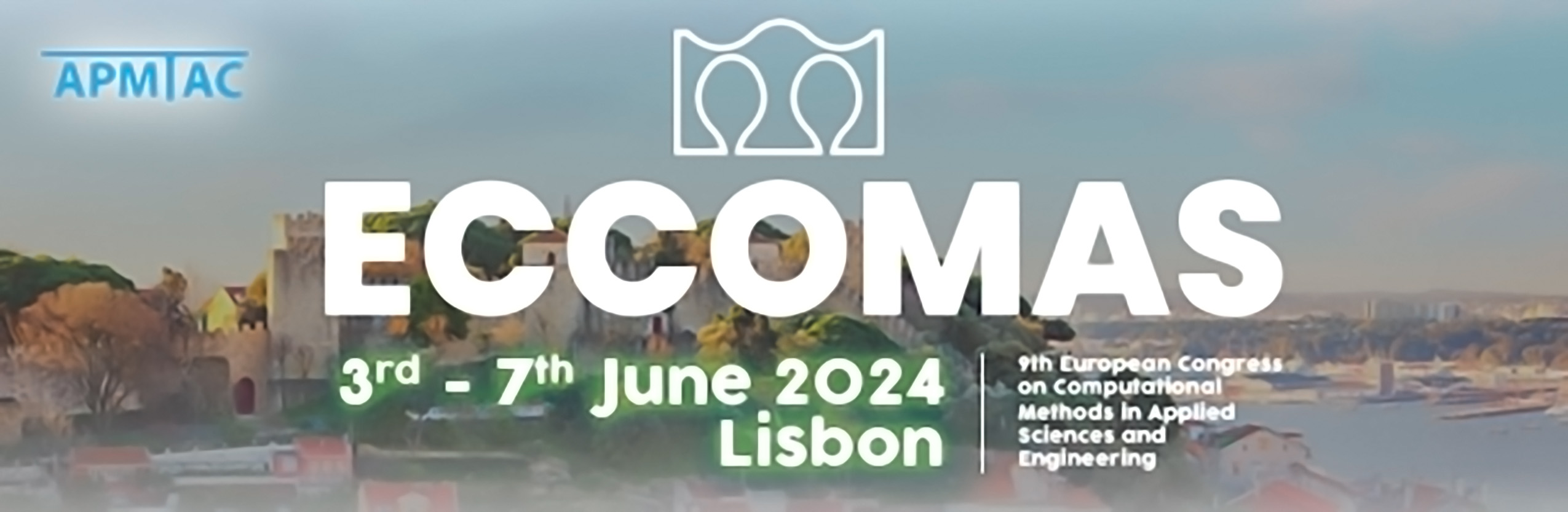
Uncertainty quantification of time-average quantities of chaotic systems
Please login to view abstract download link
The performance of real-world systems is significantly affected by the uncertainty of the parameters that define these systems. Large research effort has focused on the quantification of the effect of such stochastic variations to a Quantity of Interest (QoI), usually a time-averaged quantity. In this presentation, we consider the effect of multiple stochastic parameters on the uncertainty of QoI’s of chaotic systems. For example, in the case of turbulent flow around an airfoil, these quantities can be the time-average lift and drag coefficients, and the stochastic parameters the angle of attack, the Reynolds/Mach numbers, or the surface roughness. In order to quantify this uncertainty, we employ the recently proposed [1] sensitivity-enhanced generalized polynomial chaos (gPC) expansion, or se-gPC. This is an extension of the standard gPC expansion, where the least squares system for the evaluation of the spectral coefficients of the expansion is enriched with the sensitivity of the time-averaged quantities with respect to the stochastic variables. When all the sensitivities are computed efficiently in a single step with the adjoint method, the computational cost of se-gPC scales as m^(p-1) (where m is the number of stochastic parameters and p is the polynomial order), instead of m^p as in the standard least squares method. Therefore, se-gPC becomes increasingly useful as the number of stochastic inputs grows. Efficient sampling algorithms are also employed to reduce further the number of required evaluations [1]. For the special case of first order spectral representation, i.e. for p=1, the gPC spectral coefficients can be estimated with a single direct and a single adjoint evaluation, regardless of the number of stochastic inputs. To compute the sensitivities for chaotic systems, the adjoint of the least-squares shadowing operator, introduced in [2], is derived in the frequency domain. The method is applied to the Kuramoto-Sivashinsky equation, a well-known chaotic system, and is found to produce results that match very well with Monte-Carlo simulations. The efficiency of the proposed method significantly outperforms sparse-grid approaches, like Smolyak Quadrature. These properties make the method suitable for application to other dynamical systems with many stochastic parameters. REFERENCES [1] K. Kantarakias and G. Papadakis (2023), J. Comp. Physics, vol 491, 112377. [2] K. Kantarakias and Papadakis G. (2023) J. Comp. Physics, Vol. 474, 111757.