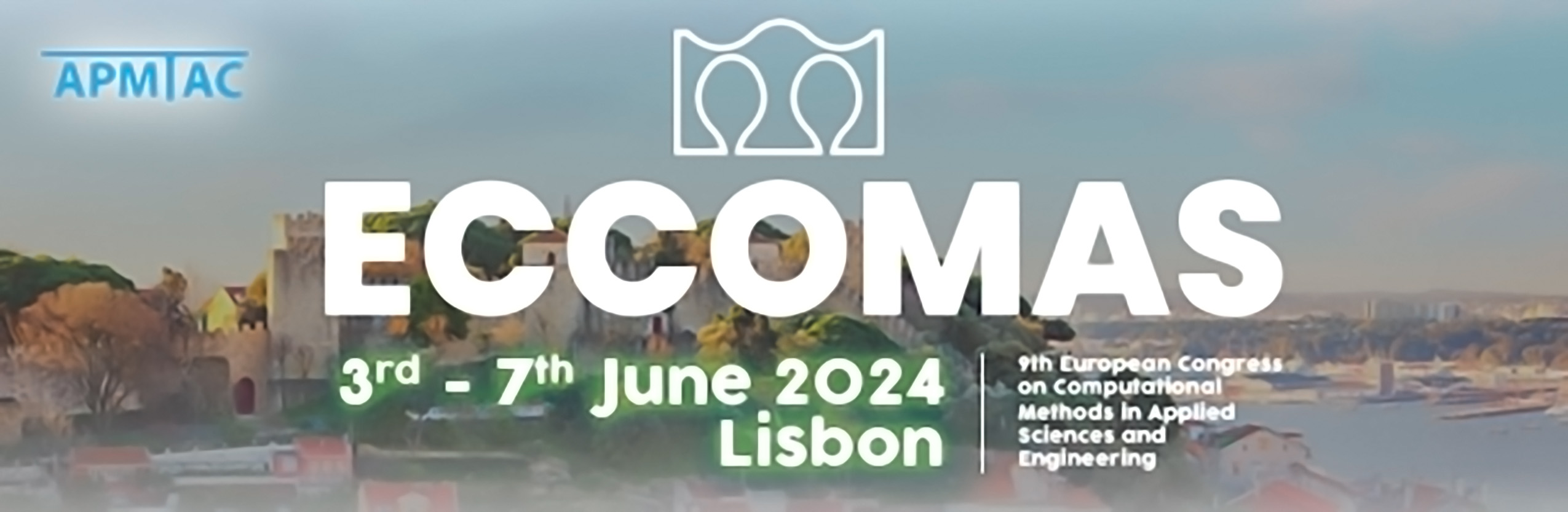
Integrating Dimensionality Reduction and Bayesian Regression in a Reduced-Order Modeling Framework for Atmospheric Boundary Layer Flows Simulations
Please login to view abstract download link
Effectively understanding and predicting atmospheric boundary layer (ABL) flows in urban settings is critical across various applications. However, this task is intricate, given the multitude of uncertain parameters and the vast computational domains involved. Computational Fluid Dynamics (CFD) has become a crucial tool for understanding the physical processes in urban flows. Nevertheless, the computational expenses linked to thoroughly investigating pertinent operating conditions and modelling parameters pose an obstacle for practical issues, such as predicting air quality in urban environments, given the constraints of current tools and resources. The current work proposes a pragmatic solution through the adoption of reduced-order models (ROMs). By leveraging ROMs, we can unravel the underlying physical processes in ABL flows with efficiency, offering valuable insights while significantly reducing the computational burden. The aim of this study is to explore the potential application of an innovative Reduced-Order Modelling (ROM) framework for fast and accurately estimating urban flows. This approach integrates dimensionality reduction techniques, specifically Principal Component Analysis (PCA) and autoencoders, along with non-linear regression employing Gaussian Process Regression (GPR). The methodology is exemplified through the analysis of flow and dispersion around a wall-mounted building and an array of buildings from the CEDVAL database. A series of training simulations is generated using a design of experiments (DoE), defining a realistic range of variables concerning the nominal Atmospheric Boundary Layer (ABL) data. The numerical simulations utilize an ABL turbulence modelling framework based on k-omega.