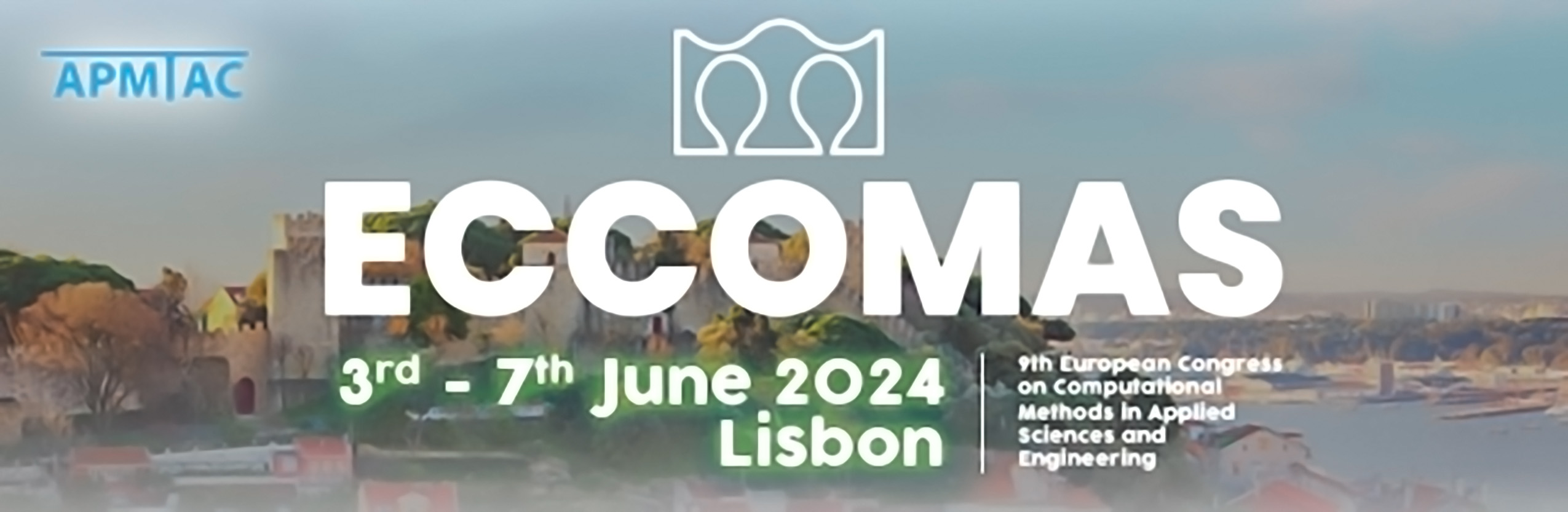
On the Role of the Fiber in Model Reduction
Please login to view abstract download link
Recent advances enable accurate forecasting of nonlinear dynamical systems on low-dimensional curved manifolds learned from data. The use of curved manifolds has proven critical in applications to high Reynolds number fluid flows where advecting flow structures are poorly approximated in low-dimensional (flat) subspaces. We show that choices of low-dimensional modeling variables that faithfully embed the underlying manifold can still lead to poor forecasting performance when states are condensed along fibers that fail to properly account for fast dynamics and transient amplification mechanisms associated with non-normality. To illustrate, we consider a non-parallel complex Ginzburg-Landau equation in a regime following a supercritical Hopf bifurcation. We project onto the unstable manifold and compare various choices for the projection fiber. We show that accurate models can be obtained by removing the most quickly decaying eigenmodes, which are orthogonal to the most slowly decaying left eigenvectors. For systems in regimes far away from relevant equilibria, low-dimensional variables with guaranteed forecasting ability can be extracted using the recently introduced Covariance Balancing Reduction using Adjoint Snapshots (CoBRAS) method and its nonlinear kernel-based variant. A common feature of successful methods based on left eigenvectors or CoBRAS is the reliance on adjoint-based sensitivity analysis. In the absence of additional physics-based knowledge providing implicit or explicit information about sensitivity, we explain why the adjoint is required to overcome the curse of dimensionality when selecting modeling variables.