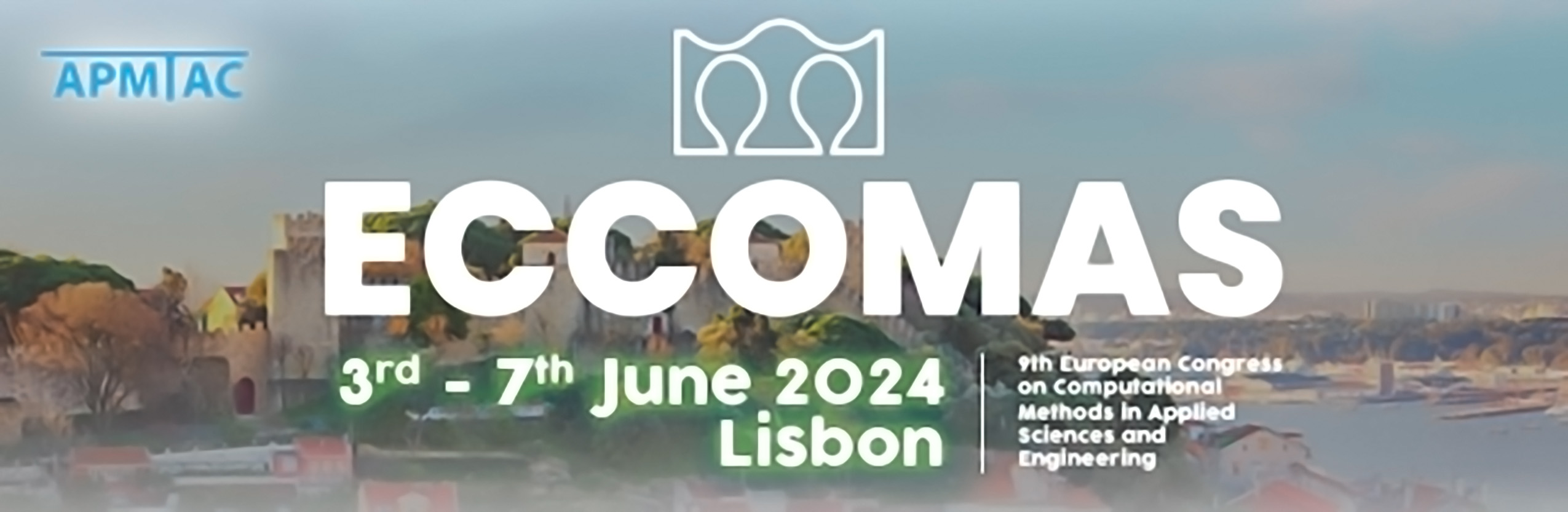
Physics-informed machine learning surrogate for applications in structural health monitoring and development of digital twins of wind turbines
Please login to view abstract download link
With increasing demand for renewable energy, wind turbine technology has evolved significantly over the last decade. Multi-megawatt wind turbines with hub heights over 120m and blade lengths more than 90m are now routinely installed. With increasing scale, the wind turbine structure is subjected to higher loads. The increase in scale also results in reduced stiffness of the wind turbine structures. The combined effect of increased loads and reduced stiffness leads to a higher magnitude of deformations and greater fatigue damage. In addition, the efficient operation of these large-scale wind turbines is essential to minimize downtime and the levelized cost of energy. Knowledge of the performance parameters of operating wind turbines is necessary for accurate damage prediction and efficient operations. However, only some of the performance parameters of an operating wind turbine can be accurately measured in practice. In addition, the installation of multiple sensors for on-site measurements leads to higher costs. In this study, the authors aim to use physics-informed machine learning surrogates to predict the critical operating parameters. The objectives of this study are: 1. Proposing an optimum sensor placement strategy using the feature importance algorithms 2. Prediction of the key operating parameters of a wind turbine using physics-informed machine learning surrogate The surrogate model developed in this study can be used for applications in structural health monitoring and the development of digital twins. This study focuses on the state-of-the-art IEA 15MW wind turbine, the largest standalone reference wind turbine with a hub height of 150m and blade length of 120m. The training data for the surrogate models is simulated using OpenFast, a widely used open-source platform for modelling wind turbines. This study develops a physics-informed surrogate model using a feed-forward neural network to predict the aerodynamic forces acting on the wind turbine blade. The input features chosen are the inflow wind speed, rotor speed, and blade pitch angle, which are routinely measured during operation. A weighted objective function is defined, which balances the data-fit objective and physics-based constraint on the output parameter. This model bypasses the computationally expensive blade element theory and provides a quick estimation of the aerodynamic forces. In addition, a sequence model is developed using a long short-term memory (LSTM) algorithm to predict t