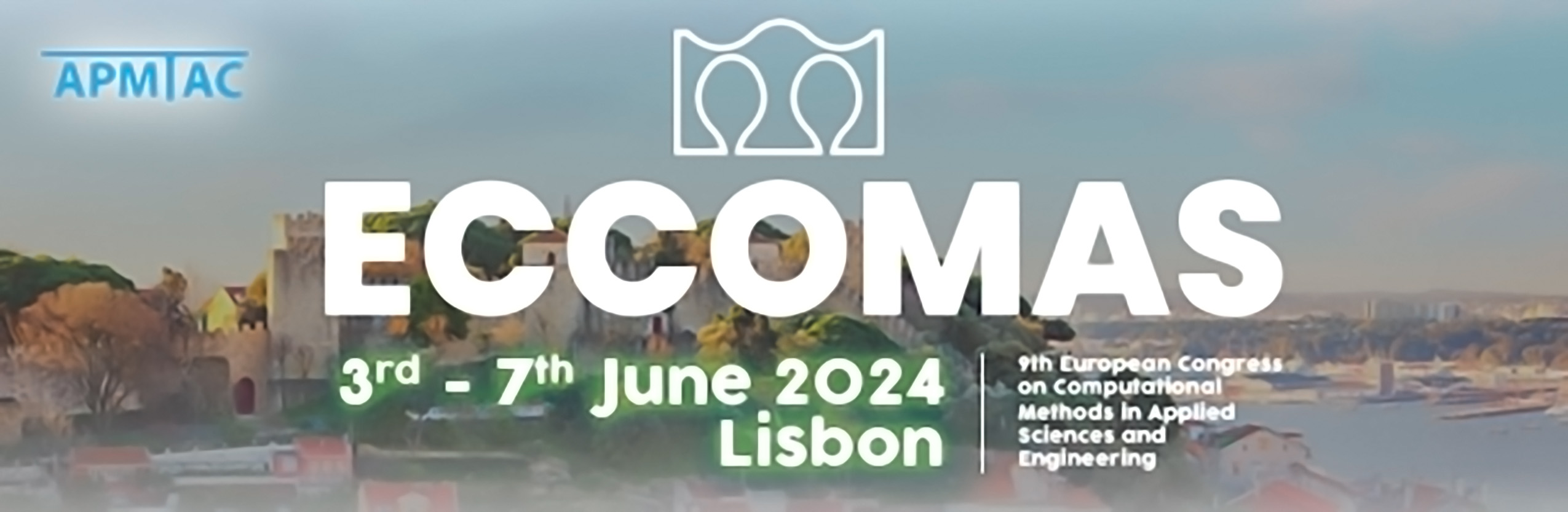
Guaranteed Stability in Inference of Nonlinear Dynamical Systems Using Quadratic Lyapunov Functions
Please login to view abstract download link
The field of learning dynamical systems is evolving rapidly, integrating data-driven modeling tools with physics-based approaches, optimization techniques, and empirical knowledge. It plays a crucial role in engineering design cycles and digital twinning. In this talk, we primarily focus on an operator inference methodology that builds preferably low-dimensional dynamical models, with a prior hypothesis on the model structure, often determined by known physics or given by experts. One of the critical properties of dynamical systems is stability. However, stability is often not guaranteed by the inferred models. Our primary focus in this work lies in developing a method that allows for the inference of nonlinear dynamical systems while ensuring guaranteed stability. To this aim, we make use of quadratic Lyapunov functions, which provide a certificate of stability. By combining the structure of nonlinear dynamical systems and the existence of Lyapunov functions, we are able to propose parametrizations of stable nonlinear models. Those parametrizations are then used to learn jointly the system's operators and the associated Lyapunov function. This framework not only enhances the accuracy of system inference but also ensures stability throughout the learning process. We present several numerical examples, illustrating the preservation of stability and discussing its comparison with the existing state-of-the-art approach to infer operators.