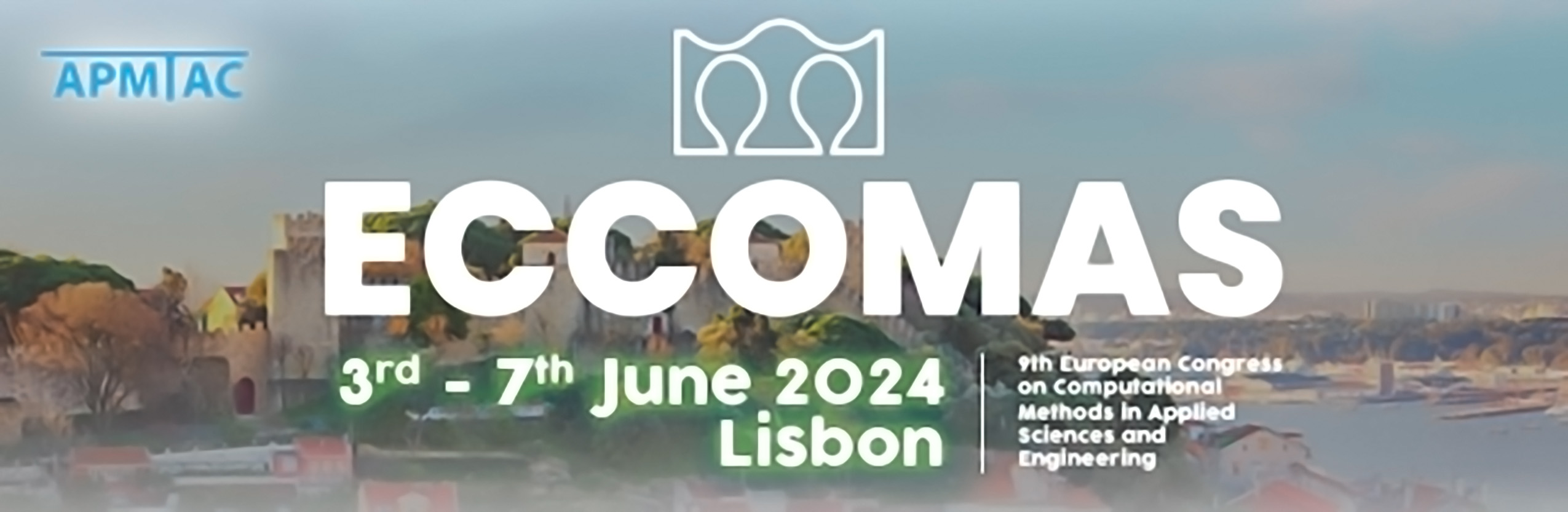
Hyperparameter Optimization for Causal Marching Physics Informed Neural Network for Hyperelasticity
Please login to view abstract download link
Millions of people suffer from traumatic brain injury (TBI) every year [1]. Inspired by Raissi et al.’s Physics Informed Neural Networks (PINNs) concept in 2019, our goal was to create a real-time, fully connected dense multilayer perceptron to model homogeneous deformation in soft solids, such as the brain [2]. This work presents a mesh-free physics-informed neural network (PINN) model for higher-order hyperelastic material models. Trained without labeled data, our PINN employs an innovative incremental-training algorithm to capture the causality of homogeneous deformation. Utilizing non-dimensionalized governing equations and minimizing residuals, our approach ensures efficient training [3]. The developed PINNs model accurately captures three-dimensional hyperelasticity, particularly for high-order models and various homogeneous deformations. Once trained, the model promptly responds to any spatial coordinate within the physical domain for the specified deformation. The data-free PINNs solution for the Landau hyperelastic model demonstrated low RMSE values of 0.04%, 0.08%, 0.16%, 0.18%, 0.07%, and 0.10% for uniaxial tension (UT), uniaxial compression (UC), biaxial tension (BT), biaxial compression (BC), pure shear (PS), and simple shear (SS), respectively. These results confirm the effectiveness of the proposed approach for various test cases. REFERENCES [1] B. B. Tripathi, D. Esp ́ındola, and G. F. Pinton, “Piecewise parabolic method for propagation of shear shock waves in relaxing soft solids: One-dimensional case,” International Journal for Numerical Methods in Biomedical Engineering, vol. 35, no. 5, pp. 1–17, 2019. [2] M. Raissi, P. Perdikaris, and G. E. Karniadakis, “Physics-informed neural networks: A deep learning framework for solving forward and inverse problems involving nonlinear partial differential equations,” Journal of Computational Physics, vol. 378, pp. 686–707, 2019. [3] G. Kissas, Y. Yang, E. Hwuang, W. R. Witschey, J. A. Detre, and P. Perdikaris, “Machine learning in cardiovascular flows modeling: Predicting arterial blood pressure from non-invasive 4D flow MRI data using physics-informed neural networks,” Computer Methods in Applied Mechanics and Engineering, vol. 358, p. 112623, 2020.