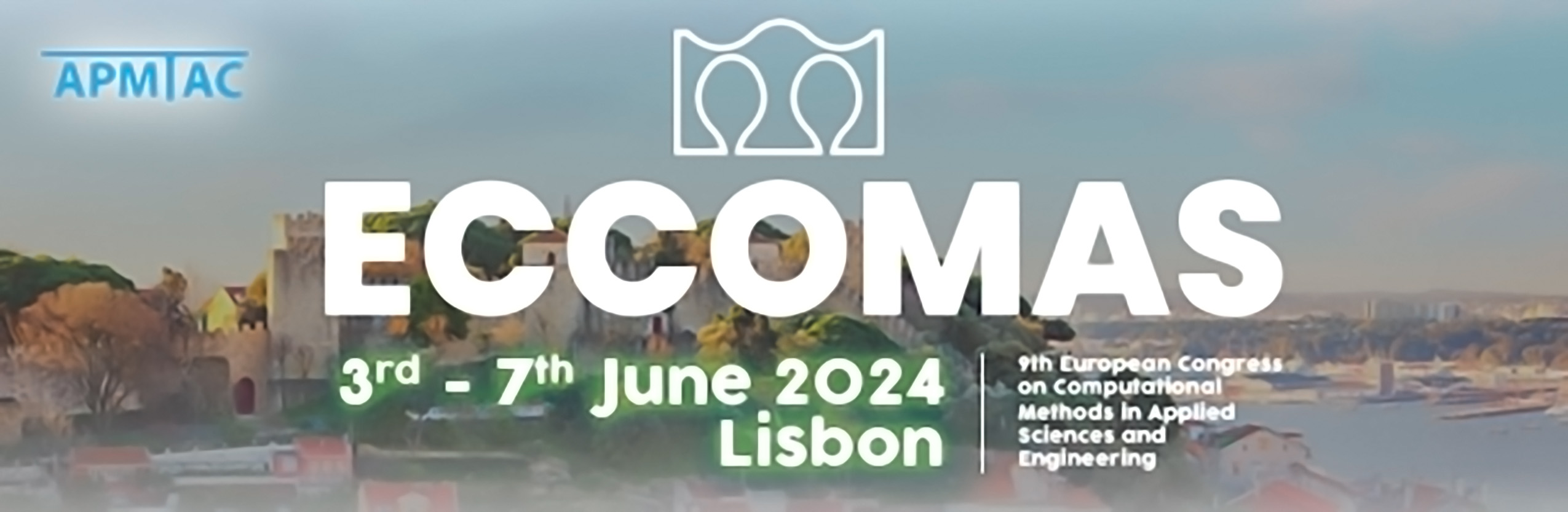
Data-Driven fast urban flow predictions featuring extreme events
Please login to view abstract download link
Having real-time and accurate numerical predictions of urban wind flow can be extremely useful for developing tools intended to improve citizens’ life quality and health. However, traditional methods such as Computational Fluid Dynamics (CFD) are unsuitable for fast prediction. This work proposes using Convolutional Neural Networks (CNN) trained with a newly created vast dataset to enable fast and accurate flow predictions for any urban geometry. The dataset has been generated through high-fidelity CFD simulations of 30 different European Urban areas and 90 meteorological conditions. The geometries were selected to have a wide variety of urban flow patterns and geometrical features, allowing the Neural Network (NN) to learn a representative range of urban flow conditions. Then, a CNN was trained to reproduce the urban wind flow for any urban geometry and meteorological condition. Preliminary results showed a significant overrepresentation of wind speed values around the spatial average. Standard loss functions used in deep learning architectures such as mean square error or mean absolute error, tend to overrepresent mid-range values at the expense of the extreme ones. In the particular case of urban flow prediction, these wind speed peaks are relevant since they significantly impact pedestrian comfort. In order to overcome this limitation, the Squared Error-Relevance Area loss function [2], specific for extreme value optimized prediction, has been applied to improve the neural network’s ability to predict the whole range of wind speeds throughout the urban geometry. The overall strategy allows for predicting accurate time-averaged wind flow in urban areas that have not been seen in training time, showing good generalization properties.