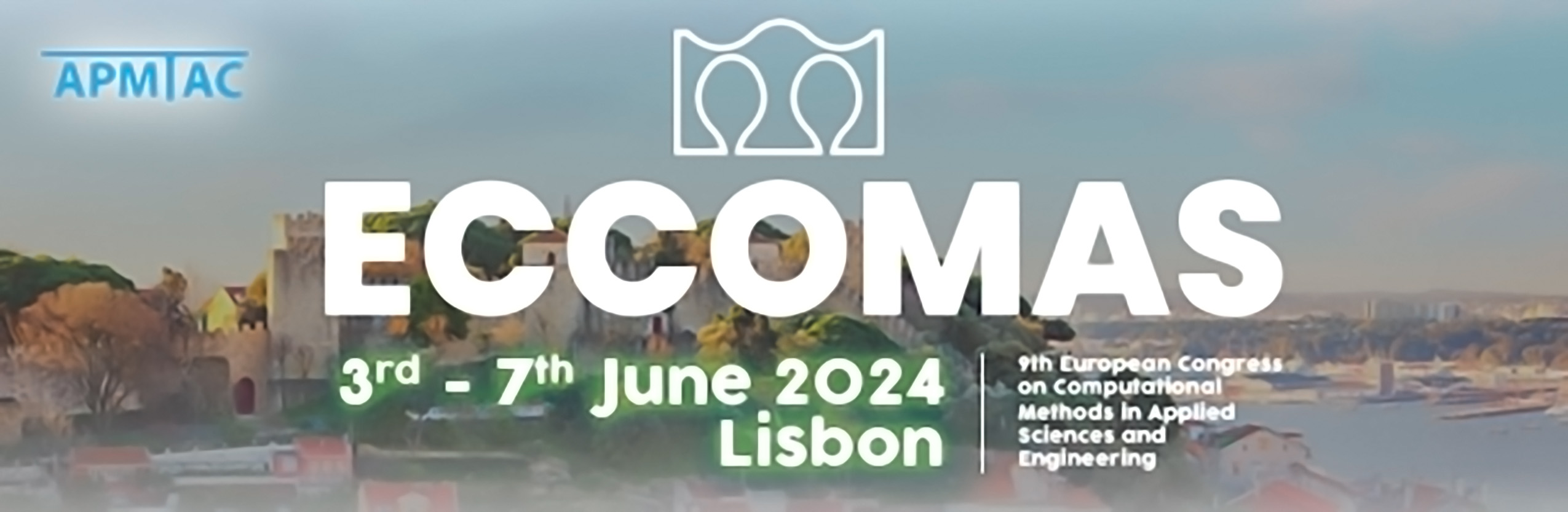
Progressive construction of projection-based ROMs for data assimilation
Please login to view abstract download link
We present an adaptive on-the-fly training strategy for projection-based reduced-order models (ROMs) to accelerate the solution to optimization problems, with emphasis on parameter estimation (calibration) of constitutive laws in structural mechanics. Our approach leverages (i) multi-fidelity training based on hierarchies of reduced-order and full-order models; (ii) incremental data compression and hyper-reduction strategies to reduce the cost of updating the ROM during the optimization loop; (iii) a trust region method to effectively combine models of different accuracy. We illustrate the effectiveness of our method through a range of synthetic model problems in nonlinear steady and unsteady structural mechanics.