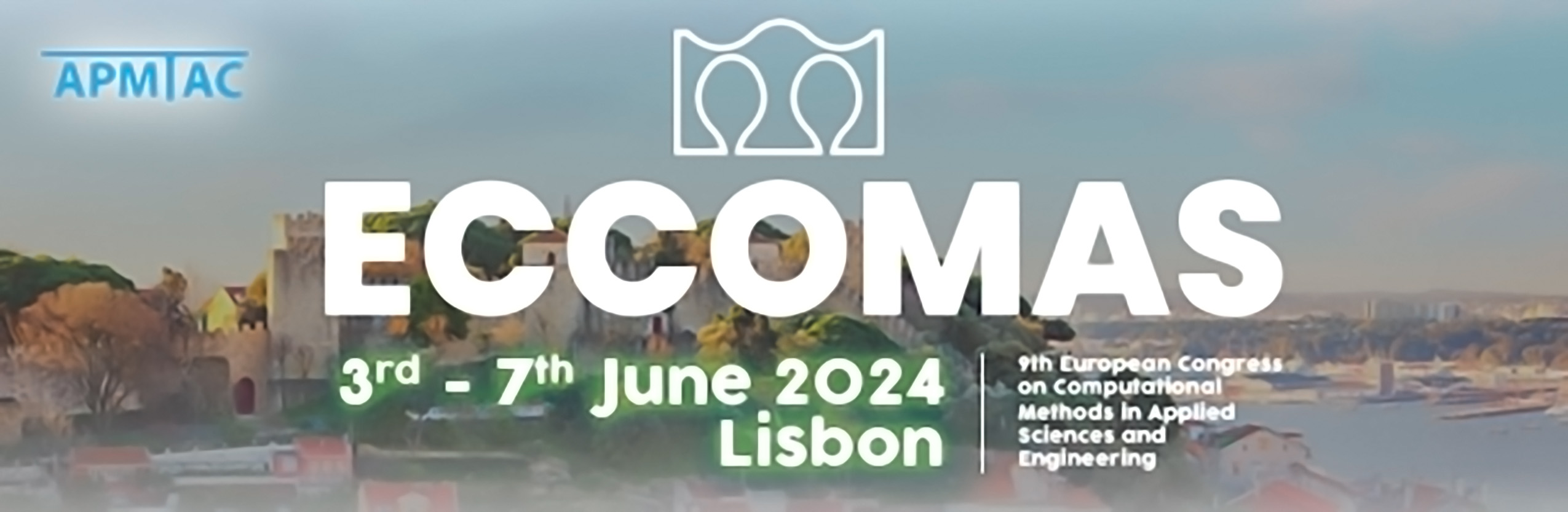
Original Eigenstrain Framework Combined with Machine Learning for Fast Residual Stress and Distortion Prediction in LPBF Parts
Please login to view abstract download link
Laser powder bed fusion (LPBF) is a popular additive manufacturing process for creating intricate metal components. However, this process is notorious for generating residual stresses that can cause distortion and cracking of the part, which poses a significant challenge to the desire of first-time-right high-quality manufacturing. High-fidelity thermomechanical simulations are an effective way to analyse residual stress development and optimize LPBF process conditions to ensure the quality of the final product but are restricted to very small domains due to the computational cost. To enable simulation of residual stresses and distortion for real-size LPBF components, approximate alternatives such as the inherent strain method (IS) have been proposed. Besides the known limitation of the method in terms of accuracy, the duration required for a single run based on IS spans hours or even days and is suboptimal for the efficient design and optimization process of components manufactured through LPBF. This research activity aims to extend the applicability of the original eigenstrain framework for LPBF. Instead of applying a homogenized inherent strain tensor in a layer-wise fashion (as done in the IS method for LPBF), artificial neural networks (ANN) are trained and employed to approximate the distribution of the final inhomogeneous eigenstrain field (i.e. the sum of all incompatible permanent strains that are the cause for the residual stresses), for the entire component. Accordingly, the residual stress state and distortion is calculated through a single liner elastic finite element calculation. In this study, eigenstrain fields of a set of geometries were calculated through the block dump method and used for training the ANN. Performance assessment of the established machine-learning assisted (MLA) eigenstrain method was conducted on unseen geometries for the ANN. The MLA eigenstrain method eliminates the need for layer-by-layer calculations compared to the IS method for LPBF and brings new opportunities for design and optimization procedures for real-size LPBF components through a drastic reduction in the computational cost.