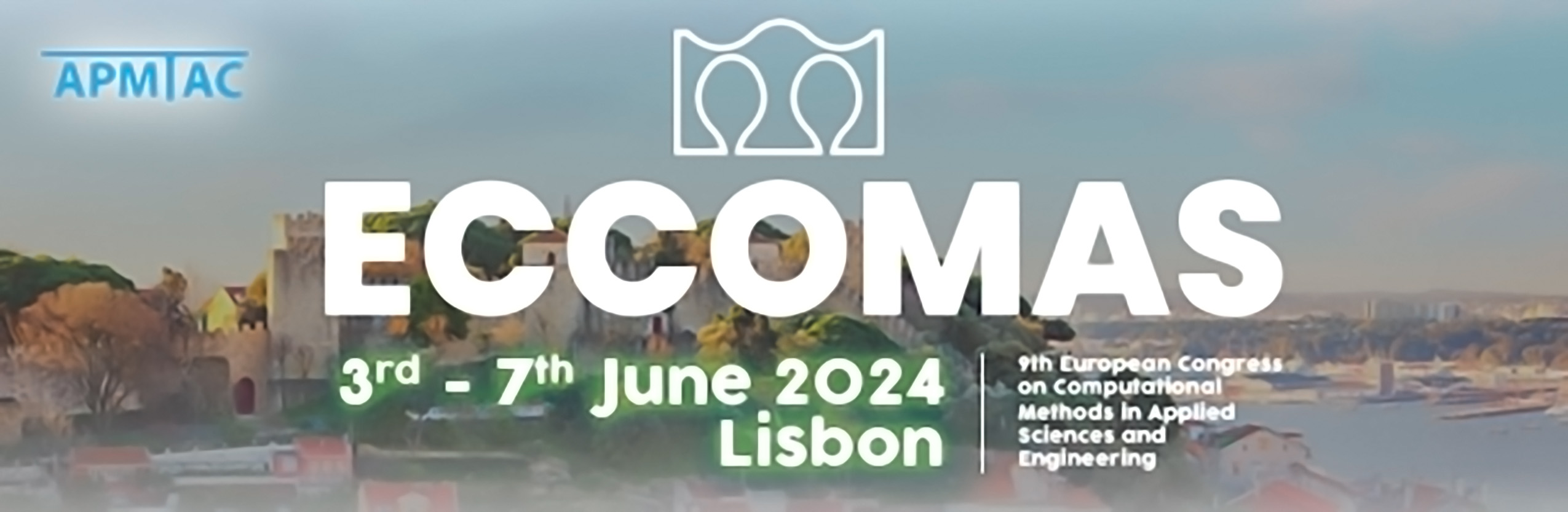
Spatio-temporal Parallel Physics-informed Neural Networks: A Framework to Solve Inverse Problems in Fluid Mechanics
Please login to view abstract download link
Physics-informed neural networks (PINNs) are widely used to solve forward and inverse problems in fluid mechanics. However, for cases with large spatio-temporal domains and/or high Reynolds numbers, the current PINNs framework suffers from extremely difficult hyper-parameter tuning and prohibitively long training time. In order to overcome these issues and enhance PINNs' efficacy in solving inverse problems, this paper proposes a spatio-temporal parallel physics-informed neural networks (STPINNs) framework that can be deployed simultaneously to multi-Central Processing Units (CPUs). The STPINNs framework is specially designed for the inverse problem of fluid mechanics by utilizing an overlapping domain decomposition strategy and incorporating Reynolds-averaged Navier–Stokes equations, with eddy viscosity in the output of neural networks. The performance of the proposed STPINNs is evaluated on three turbulent cases: the wake flow of a two-dimensional cylinder, homogeneous isotropic decaying turbulence, and average wake flow of a three-dimensional cylinder, and successfully reconstructed all three turbulent-flow cases with sparse observations. Our results, along with strong and weak scaling analyses, demonstrate that STPINNs can accurately and efficiently solve turbulent flows with comparatively high Reynolds numbers.