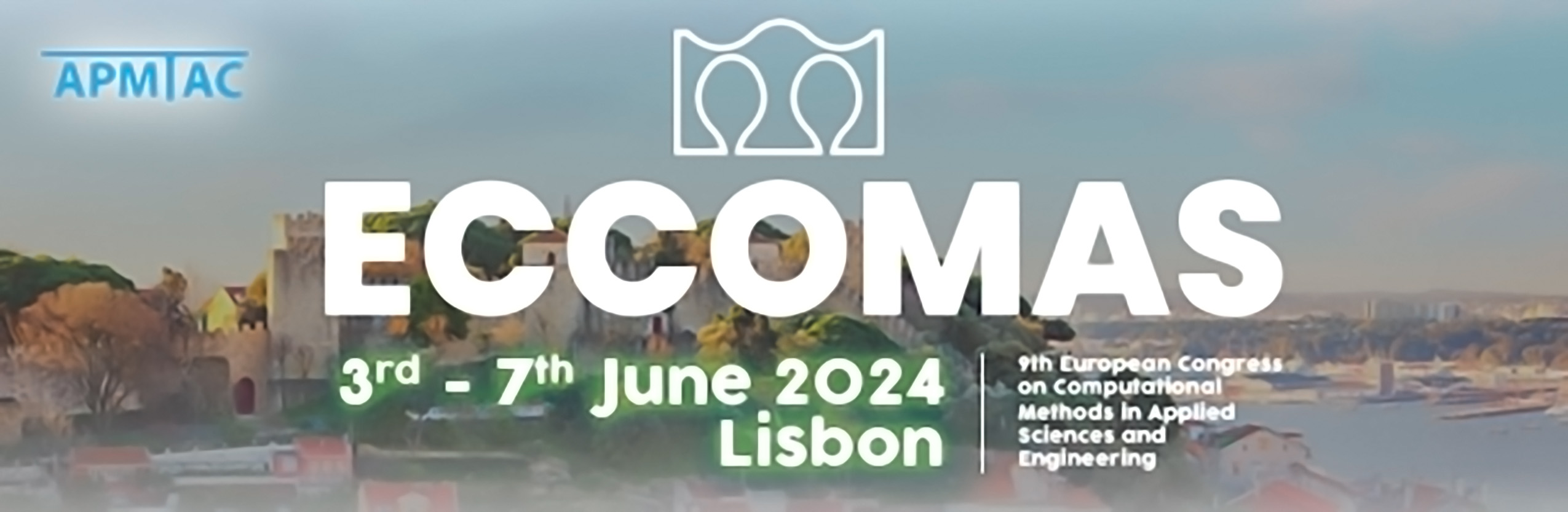
Development of ground motion models for peak ground acceleration (PGA) and acceleration spectrum intensity (ASI) using Catboost algorithm and Covariance Matrix Adaptation Evolution Strategy
Please login to view abstract download link
Peak ground acceleration (PGA) is one of the fundamental intensity measures frequently employed for a variety of purposes in the field of earthquake engineering, including structural design, damage analyses of buildings and infrastructure, seismic risk, and hazard analyses. Acceleration spectrum intensity (ASI) is the parameter related to the energy of the earthquake that has not been emphasized as much as PGA but has a strong correlation, especially with the response of the masonry buildings. This study focuses on developing an advanced nonparametric ground motion model (GMM) to predict PGA and ASI using CatBoost, a state-of-the-art machine learning (ML) algorithm. The Covariance Matrix Adaptation Evolution Strategy optimization technique is applied in the hyperparameter tuning process to robust the prediction ability of the model. Moment magnitude (Mw), focal depth (FD), shear-wave velocity averaged in the top 30 m of soil (Vs30), style-of-faulting (SoF), and Joyner-Boore distance (RJB) are utilized as estimator parameters in the prediction models. The dataset consists of 138 real earthquakes that occurred in Turkiye, Greece, and Italy, with Mw ranging from 4.4 to 7.8. Earthquakes with depths between 2.5 and 22.9 km were recorded by 843 stations, with RJB less than 500 km and Vs30 varying between 90 m/s and 1746 m/s. ML-based GMM is assessed using statistical performance metrics, including mean absolute percentage error (MAPE), correlation coefficient (R), and coefficient of determination (R2). The model indicates robust predictive abilities for PGA and ASI as evidenced by performance metrics (R≈0.87 and R2 ≈0.74).