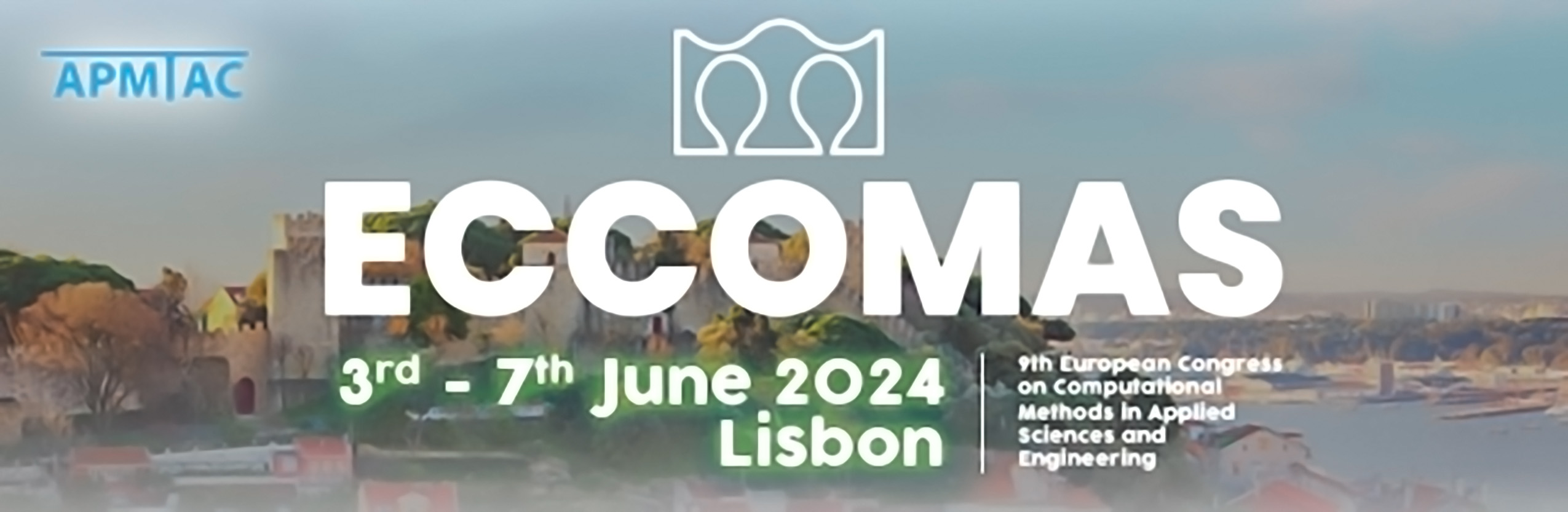
Approximative optimal experimental design in Bayesian inversion
Please login to view abstract download link
The approximation problem in Bayesian inverse problems (BIPs) has been studied intensively in the last decade. In this work, we study some stability properties of the expected utility function for the optimal experimental design in BIPs. This is the ``upstream'' problem to inverse problems, which deals with how to design the experiments to acquire the data efficiently for the inference task. We provide a framework for this problem in a non-parametric setting and prove a convergence rate of the expected utility with respect to a likelihood perturbation. This rate is uniform over the design space and its sharpness in the general setting is demonstrated. To make the problem more concrete we proceed by considering non-linear Bayesian inverse problems with Gaussian likelihood and verify that the assumptions set out for the general case are satisfied and regain the stability of the expected utility with respect to perturbations to the observation map. Theoretical convergence rates are demonstrated numerically in different examples.