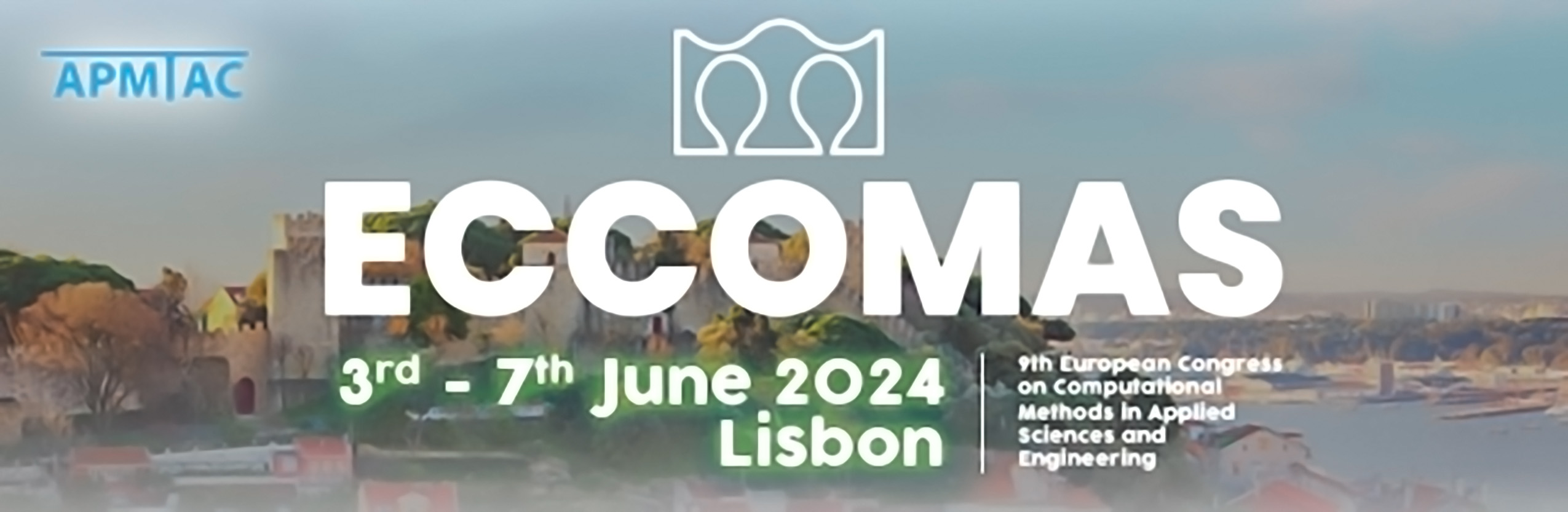
Data-Driven Reduced Models Using Radial Basis Functions
Please login to view abstract download link
Data-driven physics models, particularly for problems set in high dimensions, often suffer from the requirement to place collocation points in advance: collocation limits the approximation and optimization errors of the underlying model and necessitates a good placement of the initial basis used for the reduced-order model (ROM). Instead, we pose a data-driven ROM via a Gaussian radial basis function approximation scheme, where the shape parameters of the Gaussians are informed by data. Our approach rests fundamentally on the observation that products of Gaussian RBFs and their gradients are integrable as polynomial moments of a product distribution with a closed-form expression [1], allowing us to assemble mass and stiffness matrices in a Gaussian basis without any quadrature error. This straightforward observation enables a meshfree PDE discretization requiring no numerical quadrature, making it suitable for high-dimensional problems where partitioning space is infeasible. A variational problem posed in this Gaussian basis exhibits convergence as the number of Gaussian RBFs is increased. Due to the infinite support of Gaussians, boundary conditions are enforced by penalty, borrowing from Nitsche’s Method to mitigate coercivity error and to maintain the exhibited convergence rate. We demonstrate the method on a handful of PDE data recovery problems on a range of geometries, showing the convergence and flexibility of our approach. REFERENCES [1] Pereira JM, Kileel J, Kolda TG. Tensor moments of Gaussian mixture models: Theory and applications. arXiv preprint arXiv:2202.06930, 2022 Feb 14.