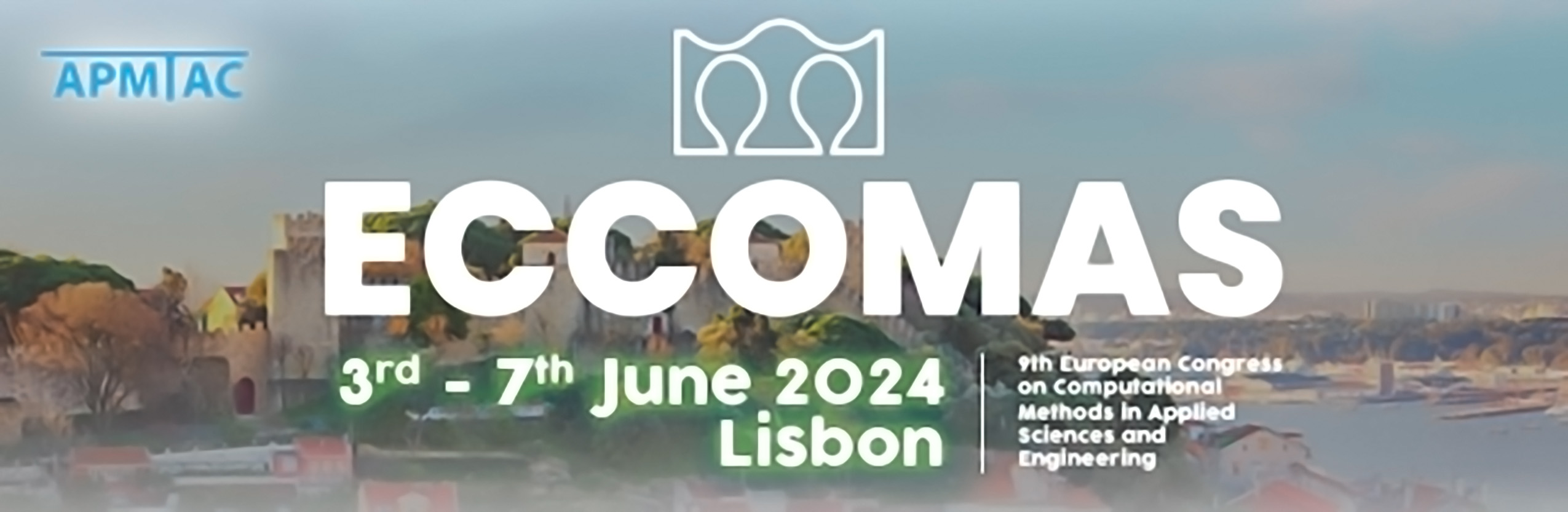
Prediction of cumulative absolute velocity (CAV) with boosting algorithms: A case study of Turkiye
Please login to view abstract download link
This study investigates the performance of Boosting Algorithms in predicting cumulative absolute velocity (CAV) of strong ground motions. In a previous effort of the authors, different machine learning (ML) algorithms, including Random Forest, Bayesian Ridge Regression, Linear Regression, Gradient Boosting, Artificial Neural Network, and Support Vector Machine were evaluated to obtain the most suitable ML-based ground motion model (GMM) for Turkiye and concluded that the best ML-based CAV GMM for Turkiye is the model obtained with the Gradient Boosting algorithm. Hence, the performance of Boosting algorithms in predicting CAV is decided to be investigated in detail. Gradient Boosting, AdaBoost, CatBoost, Natural Gradient Boosting, Light Gradient Boosting Machine, and XGBoost algorithms are utilized to obtain a superior Turkiye-specific GMM for CAV with an extended dataset. ML-based models are constructed using the vast Turkish Strong Motion Database, which has over 23,000 recordings of 743 Turkiye earthquakes. To improve the efficacy of CAV prediction, additional recordings, including the most recent 2023 earthquakes in Turkiye with Mw >6.3, are added to the dataset. Moment magnitude (Mw), shear-wave velocity averaged in the top 30 m of soil (Vs30), style-of-faulting (SoF), and Joyner-Boore distance (Rjb) are considered as estimator parameters. Statistical performance metrics and inter-event/intra-event residuals are utilized to assess the effectiveness of the ML-based models. The Turkiye-specific model obtained with the CatBoost algorithm demonstrates superior performance in CAV prediction, surpassing both traditional and other ML-based models in most evaluation metrics. Also, the CatBoost model showcases precision and reliability in predicting CAV comparable to other ML-based and traditional prediction models.