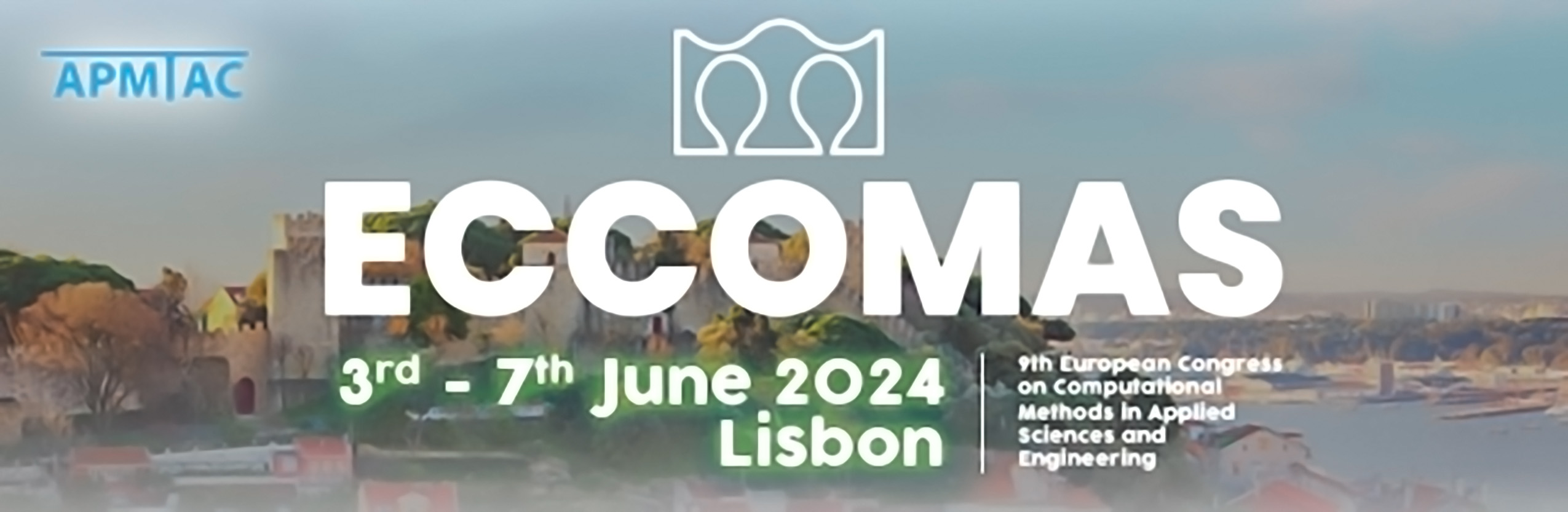
Bayesian Learning of Reduced-order Operators with Gaussian Processes
Please login to view abstract download link
This work presents a data-driven method for learning reduced-order models whose predictions are endowed with uncertainty estimates. The operator inference approach to model reduction poses the problem of learning reduced-order model operators as a regression of state space data and corresponding time derivatives. When time derivative data are not natively available, as is often the case in applications, they must be estimated from the state data with, e.g., finite difference approximations. The accuracy of the estimation greatly affects the quality of the learned reduced-order model, hence learning accurate reduced-order models in this manner is challenging when available state data are sparse or noisy. Our approach incorporates Gaussian process surrogate modeling into the operator inference framework to (1) probabilistically describe uncertainties in the state data and (2) procure analytical time derivative estimates with uncertainty. The formulation leads to a generalized least-squares regression and, ultimately, reduced-order operators that are themselves defined probabilistically. The resulting model propagates uncertainties from the observed state data to reduced-order predictions.