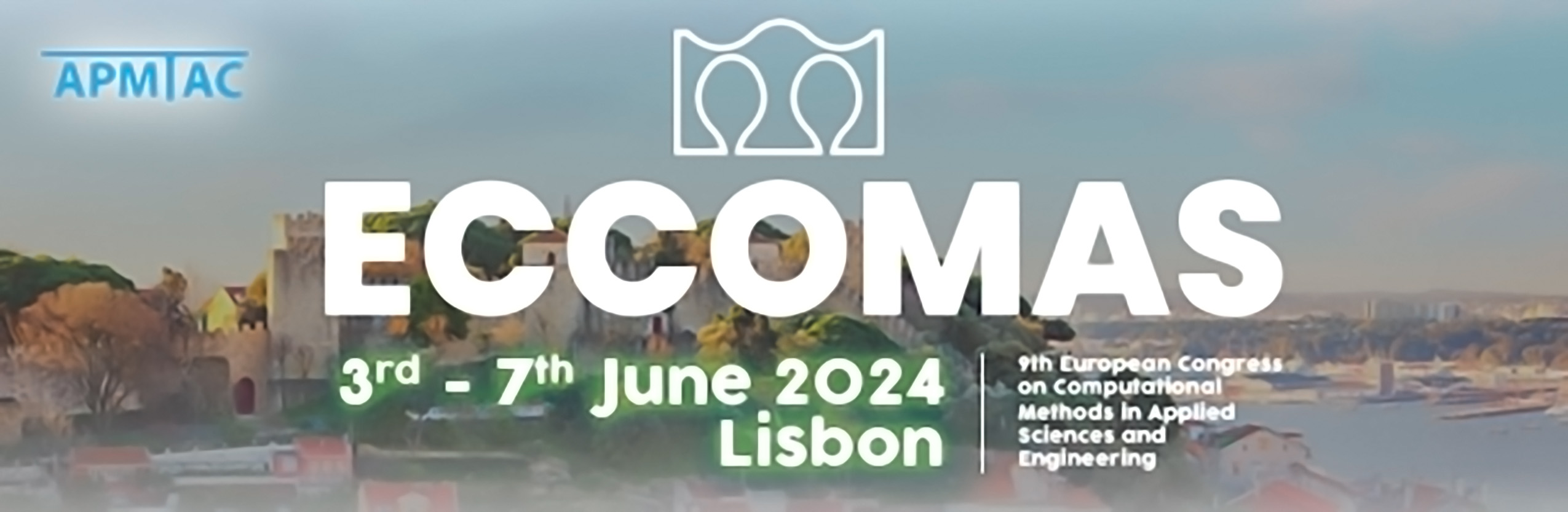
A Data-Driven Rheological Model Based on Input Convex Neural Networks
Please login to view abstract download link
In this paper, we present a data-driven rheological model based on Input Convex Neural Networks (ICNNs). ICNNs [1] are a class of neural networks that have been shown to be effective for a variety of regression tasks. In the context of rheology, ICNNs can be used to learn from a dataset of experimental data the mapping between strain and effective viscosity. Based on the theoretical analysis presented in [2], we show that the data-driven ICNN rheological model satisfies the required assumptions for the well-posedness of the associated non-linear Stokes problem. Moreover, a perturbation result can be obtained to quantify the impact of the ICNN approximation on the convergence properties of the finite-element discretization of the problem. We also present numerical results that support the theoretical estimates. We discuss the potential advantages of the ICNN approach over standard rheological laws. In particular, the traditional approach to rheology requires the a-priori selection of a specific viscosity law (power, Cross, Carreau, Carreau-Yasuda, ...), whose parameters are then fitted on available experimental data. The ICNN approach avoid this a-priori model choice and can be directly applied to new datasets. Moreover, the model can be naturally be adopted to describe complex rheological behaviors that may be difficult to capture with traditional laws. This is a joint work with A. Poiatti, J. Vené and M. Verani. [1] Brandon Amos, Lei Xu, and J. Zico Kolter, Input Convex Neural Networks, Proceedings of the Thirty-fourth International Conference on Machine Learning (ICML), 2017. [2] John W. Barrett and W.B. Liu. Quasi-norm error bounds for the finite element approximation of a non-Newtonian flow. Numerische Mathematik, 68(4), pp. 437-456, 1994.