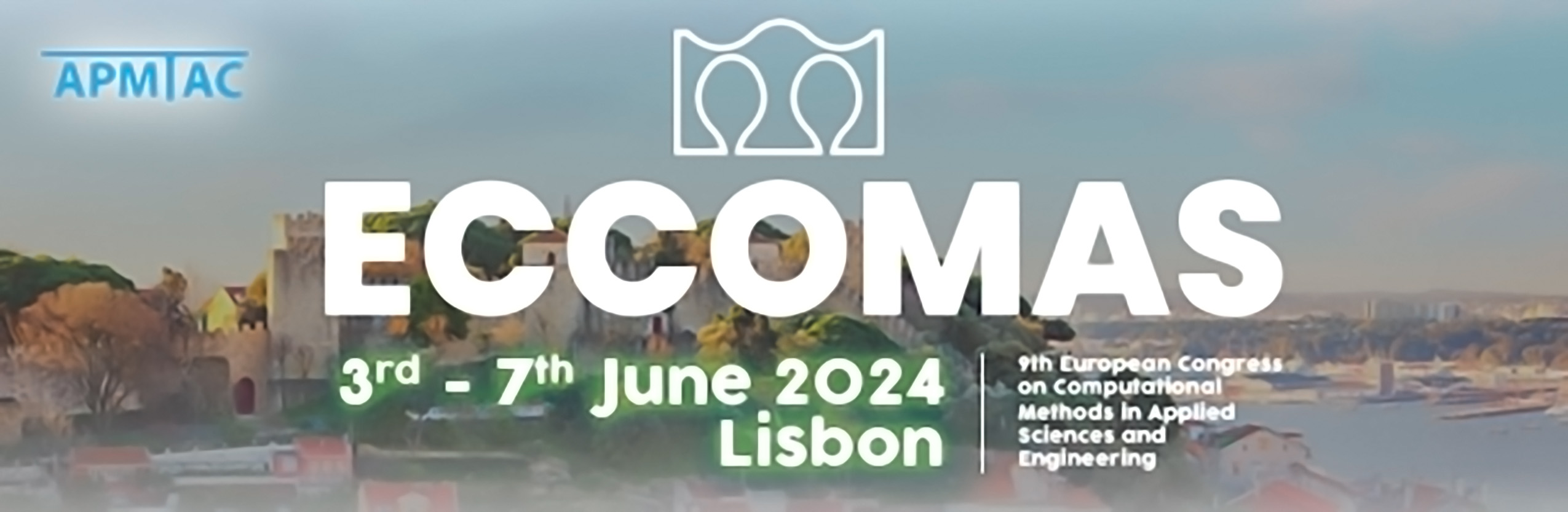
Optimization Strategies in 3D-Printed Cranial Bone Samples: Toward Accurate Identifcation of Viscoelastic Material Properties
Please login to view abstract download link
The viscoelastic material properties of cranial bone reveal a lack of data in literature within the frequency range from 100 to 10'000 Hz [1]. The structure of the human skull is of composite nature including the inner and outer cranial layer consisting of cortical bone and the diploë, the porous mid layer of cancellous bone. \\ Traditional methods for acquiring such data, like micro-indentation tests [2] or dynamic mechanical analysis, are costly and difficult to implement in this frequency range due to inherent dynamics of the measurement setup or the impossibility to measure at varying temperatures. Further, doing micro-indentation tests of porous layers is challenging due to the inhomogeneous structure of the porous material. \\ In contrast, vibrometric measurements offer a more efficient alternative. Using micro-computed tomographic scan ($\mu$CT) images, detailed geometries can be segmented and the porous layer can be homogenised. In the work of [3], the authors developed a material identification scheme for optimizing the elastic material properties of the separate domains of cortical and diploë based on fitting vibrometric experiments of dry samples with layered finite element models. However, the crucial aspect of our study lies in the accurate modelling of the frequency response, focusing on the viscoelastic properties, an attribute that is particularly important in the presence of fluids. \\ In this work, we employ vibrometric data from measured 3D printed cranial bone replicas and develop a digital representation of the measured sample using the finite element method (FEM). The viscoelastic material properties are fed as input to the FE model and allow alignment with the measured frequency response function in the range of interest. We explore both local and global optimization techniques to identify the four viscoelastic material parameters used in the fractional derivative model [4]. Additionally model order reduction and the impact of number of measured points are investigated for the most efficient and accurate solution. When applied to actual human samples, this workflow can generate crucial data to improve detailed numerical modeling of cranial dynamics, e.g. for impact studies or bone conduction hearing aids.