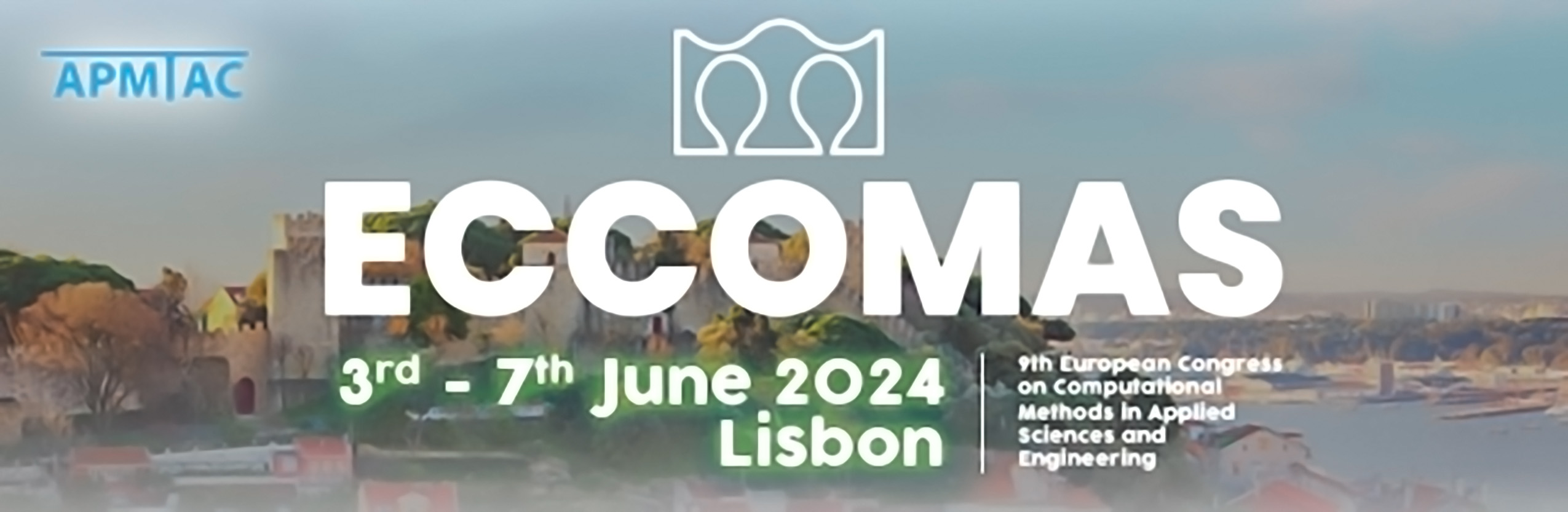
Identification of Cracks from Experimental Modal Data Using Sparsifying Priors
Please login to view abstract download link
Computer models of civil structures like wind turbines, dams, bridges etc., find their use in predicting the structure’s behaviour under different loading conditions. The structure response might change over a period of time for the same loading conditions if some form of damage, e.g., cracks build up in the structure. A previously calibrated model thus won’t produce reliable results in this situation. The model needs to account for the damage to make reliable predictions. The goal here is to predict the location of cracks i.e., damage regions and the corresponding magnitude of damage in these regions. The response e.g., strain, eigenfrequency of a structure that has undergone damage if measured could be used to identify regions of damage in it. In order to perform this identification, the Bayesian inference is deployed. Each crack is modelled as a function that approximates the failure mechanics. A certain number of these cracks are assumed to be distributed in the domain. Their positions and their corresponding damage magnitude are put in as priors. The latter is defined as a sparsifying prior that promotes sparsity in the set of inferred parameters. Therefore, on performing the inference some of the initially assumed cracks are discarded and the remaining cracks are identified and located. The modal data providing the eigenfrequency response of a concrete slab under an increasing load is used for the inference problem. The increasing damage is marked by a decreasing eigenfrequency response of the slab. Horizontal cracks that appear as the slab undergoes damage are identified, located and their contribution to the overall damage magnitude is identified.